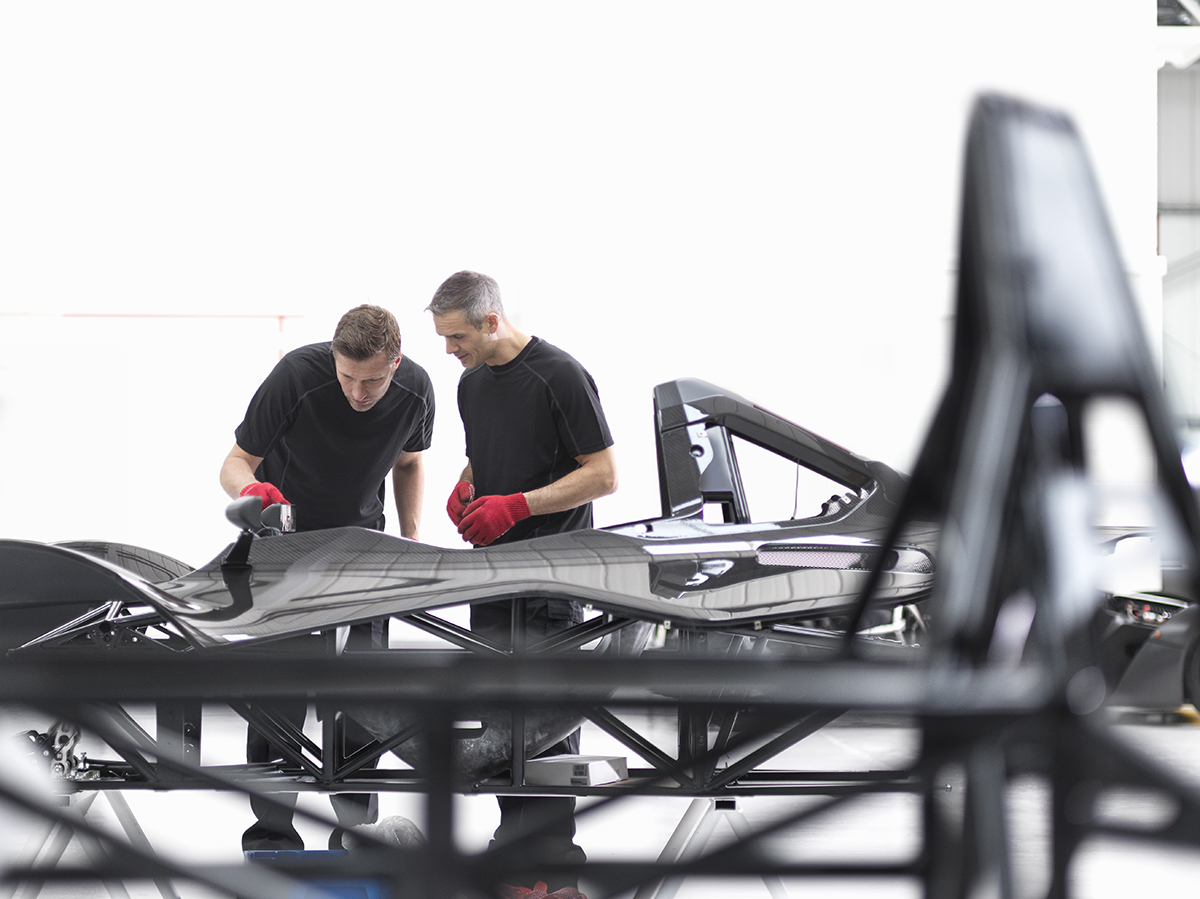
Artificial intelligence (AI) can significantly improve the accuracy and reliability of quality assurance processes, and can free up humans from dull, repetitive inspection work, letting them work on more exciting tasks. Read our blog here to find out why more manufacturers are realising the value of using AI-driven optical quality assurance systems.
However, successful AI in quality assurance depends on quality data, its management, and the implementation of the right process ecosystem, including integration in the manufacturing environment. So, there are some critical success factors that manufacturers should be aware of when implementing AI-driven optical quality assurance systems:
Number of images
Image recognition technology requires many training images so that the algorithm can identify and distinguish between different levels of quality and types of defects. To effectively automate this process, it takes around 10,000 images per defect type.
If a manufacturer typically sees around 10 to 15 different types of errors, the sheer number of training images required makes automating this process unrealistic due to the costs and logistical challenges of securing that many images up front. This is especially true if organizations have previously been using manual inspection methods, as it could mean that very few images are already available. However, it could also mean that the organization is already at a level where only a few errors are detectable.
Organizations can overcome this by choosing an algorithm that can be trained using fewer images at a faster rate. Rather than using an empty network, the method of choice is to use a pre-trained neural network. As it already contains basic observation capabilities, the network will only need 70-100 images per defect class to learn from.
This makes training the algorithm much faster and easier, and it means that the algorithm can keep learning and improving over time.
Data collection and algorithm training
It’s essential to have strong management and operational processes to govern the deployment of AI in optical inspection, especially for environments that will deploy non-AI experts in this area.
For example, a common challenge when using AI in the quality control process is the need to train the algorithm to perform the work. There are significant barriers in collecting enough training data in the form of sufficient images for every defect class.
The AI algorithm needs to be trained using imported and tagged images. In this stage, classification of the images is essential and needs to be supported by powerful, graphical user interface (GUI)-driven tools that can be operated by non-experts. The inclusion of artificially altered pictures by biasing, blurring, rotating pictures helps to increase the training set if only a few pictures are available.
To achieve the right AI results, some image pre-processing can help make error detection easier. Metadata helps to better understand how multiple pictures belong together to better classify the quality of a larger object such as a car panel or wind turbine blade. This lays the foundation for grouping images representing one larger product, which is essential. Otherwise, errors may be detected but the right conclusions may not be drawn.
AI systems need to include enough images of sufficient quality and structure for AI classification to deliver the predictive efficiency to be successful for quality assurance.
Continuous improvement of the trained model
As AI proliferates throughout manufacturing companies, and manufacturers depend more on smart factory automation for quality assurance, it’s important to continue training and testing the model. New errors and new images are generated daily, so companies must look to constantly improve their training model.
During the operational phase, called inference, it’s important to automate the ingest of images from live production lines. Furthermore, a versioning scheme can let the organization track the progress in training and AI accuracy, which would then form the basis for deploying a new model version in production.
This can enrich the training process and contribute to iterative improvements.
A graphical user interface that supports non-AI experts
In most organisations, quality assurance processes are driven not by AI experts but by production experts. To maximise the chances of success with AI-driven quality assurance processes, it’s important to include a graphical user interface (GUI) that is intuitive and easy for non-AI epxerts to use. This GUI-driven process landscape must be implemented on top of the pure AI algorithms to facilitate their usage by production experts who are not necessarily AI experts.
Getting started
Manufacturers can get started with an agile and low-risk approach that delivers benefits fast. It can be difficult to accurately and immediately predict the success of such a project because there are so many variables.
It’s more useful to start with feasibility studies or proofs of concept that, on the one hand, don’t require too much financial investment and, on the other hand, allow for quick readjustments for the next steps in an agile approach.
It’s essential to choose a partner that is not just highly skilled in the areas of AI and data science but also has strong credentials when it comes to providing top quality solutions for the manufacturing industry. Having the know-how and proven methodology to implement AI-driven optical quality assurance systems in the process landscape is crucial to the success of any AI implementation.
The partner must to be able to implement a whole-of-process scheme that’s suitable for non-experts in AI to manage, rather than simply focusing on getting the right AI algorithm.
Visit Fujitsu’s Manufacturing pages to see how our smart factory solutions can help your manufacturing business gain or retain a competitive edge in a global landscape.