A Practitioner's Guide to Databricks vs Snowflake
May 8, 2023
When comparing Databricks and Snowflake across various features and capabilities, it is evident that Databricks holds a competitive edge for TCO sensitive organisations seeking a unified analytics platform that supports all their data, users and use cases. Databricks offers a comprehensive solution for data-driven organisations and offers superior performance in:
● ETL workloads;
● processing different data types;
● cataloguing and lineage;
● AI/ML ecosystem integration; and
● real-time data processing.
Databrick’s innovative Delta Share feature through the Unity Catalog enables seamless and secure data sharing without relying on traditional connectivity patterns. While Snowflake excels in specific areas such as data warehousing and ease of deployment, Databricks emerges as the better platform for organisations looking to unlock the full potential of their data and drive impactful business decisions.
Introduction
Fujitsu Data & AI, a specialist division of Fujitsu within the APAC region, works with enterprise organisations and governments to find, interrogate, and help solve the most complex data problems across Australia, New Zealand, and Asia. Our purpose is to accelerate the growth of our customers' Data Analytics and Artificial Intelligence capabilities to unlock the value within their data. We have one of the largest data engineering capabilities in Australia and are backed by Fujitsu, one of the largest ICT service providers in the world. Using industry leading specialists, we offer full breadth, end-to-end Advanced Analytics, Business Intelligence and AI capabilities. We are a Premium Databricks delivery partner and have global partnerships with Microsoft, AWS, and Google. Our strong Databricks partnership is shown through our continued success with our clients, and we have won Databricks Regional Systems Integration Partner Asia Pacific and Japan in 2021 and 2022. Utilising our extensive specialist experience, we in collaboration with Databricks, have created this article to provide a practical perspective on the differences between Databricks and Snowflake.
Macro trends and why should you care?
In today's rapidly evolving digital landscape, businesses are inundated with a deluge of data, as the proliferation of devices, applications, and networks generates an ever-growing volume of information. As a result, companies are grappling with the challenges of efficiently managing, processing, and harnessing this massive influx of data to unlock its full potential and drive strategic decision-making.
How does a company determine the most suitable tools for addressing the mounting challenges of safeguarding sensitive data, managing the ever-increasing volume, velocity, and variety of data, and fulfilling the endless requirements of data-savvy users? Ensuring the efficient handling of the diverse sets of data and optimising their outputs necessitates complete data unification in a single location, managed by a centralised team.
Numerous innovative vendors, such as Databricks and Snowflake, have emerged in response to these real-world challenges, leveraging their extensive experience and cutting-edge technologies to assist companies in effectively managing and navigating the complexities of today's data-driven landscape. Both vendors offer data platform solutions across the three main cloud providers: AWS, Microsoft Azure, and GCP.
Before we can understand the differences between these two products, we need to understand the changing needs of companies and the new landscape in which they are competing. Companies are faced with an ever-changing data landscape as data capture, analysis, and solutions drive a competitive edge. Companies need to analyse and act on the insights produced by data solutions faster to stay competitive. The speed and types of data - unstructured; semi structured; and structured data sources, need to be processed by data platforms in a secure manner to produce the results required by the business.
Who are the players
Databricks, founded in 2013 by the original creators of Apache Spark, an open source in-memory big data processing platform, were established with the mission to simplify large-scale data processing and unlock the power of big data for organisations. Over the years, the company has developed a unified data analytics platform that combines the best of data engineering, AI, and machine learning, enabling enterprises to harness the full potential of their data. By providing a robust, collaborative, and scalable environment, Databricks empowers organisations to streamline their data workflows, accelerate innovation, and drive better business outcomes. Databricks was founded on open source and have continued to produce open-source components such as ‘Delta’ format which have been widely adopted in the industry. They are also the founders of the “Lakehouse” concept bringing together traditional warehousing and AI/ML workloads into a single unified platform. As a testament to its success, Databricks has grown into a leading data and AI platform, serving a diverse clientele across various industries, and continually pushing the boundaries of what's possible with data analytics.
Snowflake, founded in 2012 by three data warehousing experts, was created with a vision to revolutionise the world of data storage and management. The founders recognised the limitations of traditional data warehouse solutions and sought to build a cloud-native, fully managed, and highly scalable data platform. Snowflake’s unique architecture, a hybrid approach to shared-nothing MPP query cluster (every node has some amount of data) and shared-disk data storage, allows for seamless scalability, improved performance, and cost-effective solutions tailored to the needs of each organisation. Over the years, Snowflake has gained widespread recognition as a leading cloud data warehouse, serving a multitude of industries and customers around the world. With a strong commitment to innovation and customer success, Snowflake continues to break new ground in data warehousing, empowering organisations to make data-driven decisions and achieve better business outcomes.
Databricks and Snowflake are both popular technologies used in the field of data analytics and processing, but they have some key differences in their features and functionalities.
1. Data warehouse vs Lakehouse: Snowflake is a cloud-based data warehouse that provides a fully managed, scalable, and SQL-based data warehousing solution. It is optimised for fast query performance and allows users to store and analyse structured and semi-structured data.
On the other hand, Databricks is a cloud-based cloud native data platform that brings the best of data warehouse and data lake together in a new category of Lakehouse and provides a unified analytics workspace for data engineering, AI, and machine learning tasks.
It is optimised for large-scale data processing and analysis, and supports a wide variety of data formats, including structured, semi-structured, and unstructured data.
2. Architecture: Snowflake provides a SaaS based platform and uses a unique hybrid architecture of shared-nothing MPP query engine (aka virtual warehouses) and shared-disk central data storage. It provides automatic scaling, caching, and storage optimisation, making it easy to scale up or down based on the workload, and is available on all major public clouds like Azure, AWS and GCP.
Databricks, a PaaS based Platform, is built on top of Apache Spark, an open-source distributed data processing framework, and runs on cloud platforms such as AWS, Azure, and Google Cloud. Snowflake is a managed service, and its architecture is known for end users. However, Snowflake's node types are unknown and do not allow customers to modify or cost-optimise, while Databricks allows complete control over compute.
Additionally, Databricks implemented fully serverless options to further enhance customer experience.
3. Data processing capabilities: Snowflake is primarily focused on providing a high-performance SQL-based data warehousing solution, with support for complex queries, transactional processing, and data integration through its ecosystem of connectors and integrations.
It also provides features such as data sharing, data replication, and data masking for improved data governance. Databricks provides a wide range of data processing capabilities beyond SQL, including real time stream processing, machine learning, and graph processing, using the power of Apache Spark.
Databricks also provides built-in libraries for machine learning and deep learning, such as MLlib and TensorFlow, making it a popular choice for AI/ML and machine learning tasks. More recently, Databricks also included the ability to build and deploy Large Language Models (LLM). Databricks now provides a fully open-sourced LLM called Dolly.
4. Collaboration and notebooks: Databricks provides both a collaborative notebook experience that is widely adopted by analysts and data scientists while also providing data engineers complete IDE integration with a growing list of popular tools like VS Code, Pycharm, etc. It provides a unified environment for data engineers, data scientists, and business analysts to collaborate and iterate on data projects.
Snowflake, on the contrary, does not provide built-in support for notebooks or collaboration features. However, users can integrate Snowflake with other tools for data visualisation, reporting, and collaboration.
5. Security and compliance: Both Snowflake and Databricks provide robust security features for protecting data and ensuring compliance with data regulations. Snowflake provides features such as data encryption at rest and in transit, role-based access control (RBAC), and auditing. It also supports features such as virtual private cloud (VPC) peering for enhanced network security.
Databricks provides similar security features, along with functions such as data lake firewall, data lake encryption, and integration with Azure Active Directory for authentication and authorisation
In terms of data ownership, Snowflake has decoupled storage and processing with ownership over both layers. However, storage is proprietary, controlled by Snowflake (and partners that Snowflake permits). Access to this storage comes at a cost to the customer. Databricks has fully decoupled storage layers and allows users to store data anywhere in any format, focussing on open standards and the freedom of choosing the processing engine while integrating with 3rd party solutions.
6. Pricing: Pricing can be complex to compare as both providers offer different pricing models and different pricing tiers. Databricks' pricing is considered more cost-effective than Snowflake due to its flexible and scalable cost structure, which better accommodates the needs of organisations of various sizes and budgets. Databricks offers a pay-as-you-go model, where customers only pay for the resources they consume, thus optimising expenses according to their workloads. Additionally, the platform provides features like auto-scaling and auto-termination, which further helps control costs by automatically adjusting resources based on usage and terminating idle clusters. Databricks also offers better pricing when it comes to ETL/ELT workloads. Running standard Spark-based clusters allow for a very flexible pricing model.
Snowflake uses a more rigid pricing model based on pre-allocated compute resources, which can result in overprovisioning and underutilisation of resources, ultimately leading to higher costs. Therefore, Databricks' pricing flexibility and resource optimisation make it a more cost-effective solution compared to Snowflake.
Recent innovations
As we dive into the world of Databricks and Snowflake, it's crucial to examine the innovative features that set these platforms apart. Both Databricks and Snowflake have consistently pushed the boundaries of data engineering and analytics, introducing cutting-edge solutions to address evolving business needs. In this section, we will explore some of the most recent advancements in both platforms, showcasing how their commitment to innovation empowers organisations to harness the full potential of their data and make data-driven decisions with confidence.
Databricks
1. Delta Lake: An open-source storage layer that brings ACID transactions, scalability, and reliability to data lakes. It enables organisations to manage the challenges of data reliability, quality, and performance for big data and AI workloads.
2. Delta Live Tables: These declarative SQL Pipelines are based on a truly streaming architecture making it easy for customers to develop and maintain extremely fast workflows to enable operational decision-making as well as take advantage of advancing IOT technology.
3. Delta Sharing: The world's first open protocol for securely sharing data across organisations in real-time, without the need for the other organisation to have Databricks. This innovation simplifies data sharing and collaboration, helping organisations unlock new insights and opportunities.
4. Unity Catalog: A unified data catalog that enables organisations to manage and discover datasets, as well as track data lineage across their Databricks workspaces. It streamlines data governance and provides greater visibility into data assets and their usage.
5. Databricks Machine Learning: A comprehensive solution that integrates popular machine learning frameworks, distributed ML libraries, and a collaborative UI. This platform aims to make it easier for data scientists and engineers to develop, train, and deploy machine learning models at scale.
6. Databricks SQL Warehouse: Designed to provide a fast, easy-to-use, and cost-effective way for data analysts to work with massive datasets using SQL. Databricks SQL integrates with popular business intelligence tools and offers features like auto-scaling and optimised query performance for a seamless analytics experience.
7. Dolly: A completely open-source Large Language Model (LLM) that exhibits high quality instruction-following behaviours that can be trained on fine-tuned datasets to meet specific needs of customers without the overhead.
Snowflake
1. Snowflake Data Cloud: A global data network that facilitates secure and governed access to a wide range of data sets, enabling organisations to share and collaborate on data more effectively. It allows businesses to break down data silos and accelerate their data-driven initiatives.
2. Snowflake Data Marketplace: A platform that provides access to a vast array of data from various providers, making it easy for organisations to discover, access, and utilise third-party data sets in real-time. It simplifies data acquisition and integration, helping businesses unlock new insights and opportunities.
3. Snowpark: A new developer experience that is intended to allow users to write code in familiar programming languages to perform complex data transformations and processing within Snowflake. At this stage it supports SQL translation and portions of python for basic ML. This innovation begins to extend Snowflake's capabilities to cater a broader range of data engineering tasks. At this stage there is no MLOps capability, and it is yet to support Pandas API or other statistical languages (Scala, Java, R).
4. Snowflake Data Exchange: A feature that enables secure and real-time sharing of data between Snowflake accounts, simplifying data sharing between organisations and facilitating seamless collaboration on data-driven projects.
5. Dynamic Data Masking: A security feature that allows organisations to define masking policies for sensitive data, ensuring that users only see the information they are authorised to access. This innovation enhances data security and helps businesses comply with data protection regulations.
Detailed Feature Comparison
The following table provides a comprehensive comparison of Databricks and Snowflake. By examining various features such as ETL/ELT workloads, data warehousing, data sharing, and more, we can gain valuable insights into their respective strengths and areas of expertise. This side-by-side analysis will help you make an informed decision when choosing the best platform for your organisation's unique requirements, keeping in mind factors like performance, integration capabilities, and cost-effectiveness.
Feature |
Databricks |
Snowflake |
Winner |
ETL Workloads |
Excellent performance with large-scale data processing using Apache Spark and Delta Lake. |
Can handle ETL operations with SQL-based transformations, but not as efficient as Databricks. |
Databricks |
Traditional Data Warehouse |
Offers Databricks SQL for warehousing but optimised for analytics & ML workloads. |
Designed specifically for data warehousing with separate compute and storage scaling. |
Snowflake |
Analytics at Scale |
Optimised analytics workloads to handle ETL Analytics and AI/ML Workloads faster and more efficiently |
Scales for traditional warehouse loads, but without advanced ML capabilities |
Databricks |
Data Sharing |
Offers Delta Sharing for sharing datasets across organisations, without the need for the other organisations to have Databricks or the need to utilise Databricks UI. |
Provides data sharing with other Snowflake accounts using secure data sharing. A reader account is available for users without licensing agreement. It must be setup by the provider and still utilises the Snowflake UI. |
Databricks |
Processing Different Data Types |
Supports various structured and semi-structured data types with Spark and Delta Lake. |
Primarily focused on structured data but can handle semi-structured data with SQL variants. |
Databricks |
Cataloguing and Lineage |
Offers Unity Catalog for managing and discovering datasets, and for tracking data lineage. |
Provides a native catalog (sharehouse) for managing and discovering datasets. |
Databricks |
UI |
Collaborative notebook-based interface for data exploration, visualisation, and analytics. |
Simple and intuitive web-based UI for querying and managing data. |
Databricks |
Data Analytics and SQL Reporting |
Optimised for advanced analytics, supports SQL reporting, and integrates with BI tools. |
Excellent SQL reporting capabilities and seamless integration with various BI tools. |
Tie |
AI/ML |
Built-in support for popular ML frameworks, distributed ML libraries, and collaborative UI. |
Until recently relied on integration with external ML platforms and libraries. The addition of Snowpark has reduced this gap and will be an interesting space to watch. |
Databricks |
Data Security and Sovereignty |
VNet Injection for network isolation, encryption at rest and in transit, RBAC, and NSGs. Data sits on a client managed network. |
Encryption at rest and in transit, RBAC, MFA, VPS for network isolation, and regional choice. Data resides on a Snowflake managed network. |
Databricks |
Costs (Data Warehousing) |
Pricing based on workspace usage, data processing, and storage. Costs can be controlled down to a node level providing flexibility for all ETL workloads. |
Pay-as-you-go pricing model with separate storage and compute costs. |
Databricks |
Unified Platform |
Has a wide range of capabilities as a complete unified platform allowing for AI/ML production workloads as well as data warehousing capabilities. |
Snowflake has strong data warehousing capabilities, whilst venturing into AI/ML it does not yet have production grade capabilities in this area. |
Databricks |
Ecosystem Integration |
Integrates well with a wide range of data processing, analytics, and visualisation tools. |
Provides connectors for various data integration, BI, and analytics tools. |
Databricks |
Scalability |
Recent scaling and performance improvements with Photon clusters, Job clusters and serverless SQL allow for seamless scaling out and up. Databricks allows flexibility in selecting nodes and the number of scale-out nodes. |
Independent scaling of compute and storage resources for seamless scalability. No options to choose the number of compute nodes and instance types. |
Databricks |
Real-time Data Processing |
Supports real-time data processing with Spark Streaming and Structured Streaming. |
Limited support for real-time data processing; better suited for batch processing. |
Databricks |
Multi-cloud Support |
Supports Azure and AWS and Google Cloud Platform. |
Supports Azure and AWS and Google Cloud Platform. |
Tie |
Ease of Deployment and Management |
Requires some configuration and management for optimal performance and resource utilisation. |
Fully managed software as a service with minimal setup and management overhead. |
Snowflake |
Compliance and Certifications |
Provides a wide range of compliance certifications, including HIPAA, GDPR, and SOC 2 Type II and FedRAMP. |
Provides a wide range of compliance certifications, including HIPAA, GDPR, and FedRAMP. |
Tie |
Considering the features outlined in the comparison table, Databricks stands out in ecosystem integration, real-time data processing and cost effectiveness, handling more than simply Data Warehousing capabilities. It demonstrates superior performance in areas such as ETL workloads, handling various data types, cataloguing and lineage, and AI/ML. On the other hand, Snowflake excels in traditional SQL-based data warehouse functions where no other analytics needs for semi- and un-structured data analysis or ML/AI are required in an organisation's strategy.
For an organisation wishing to maintain complete control of their data within their own network environment, Databricks managed VNET allows for data to remain in place and never be moved outside of the company’s own security controls and monitoring.
If your company needs help with your data to produce the results required by the business, please contact a Fujitsu Data & AI specialist now.


Editor's Picks
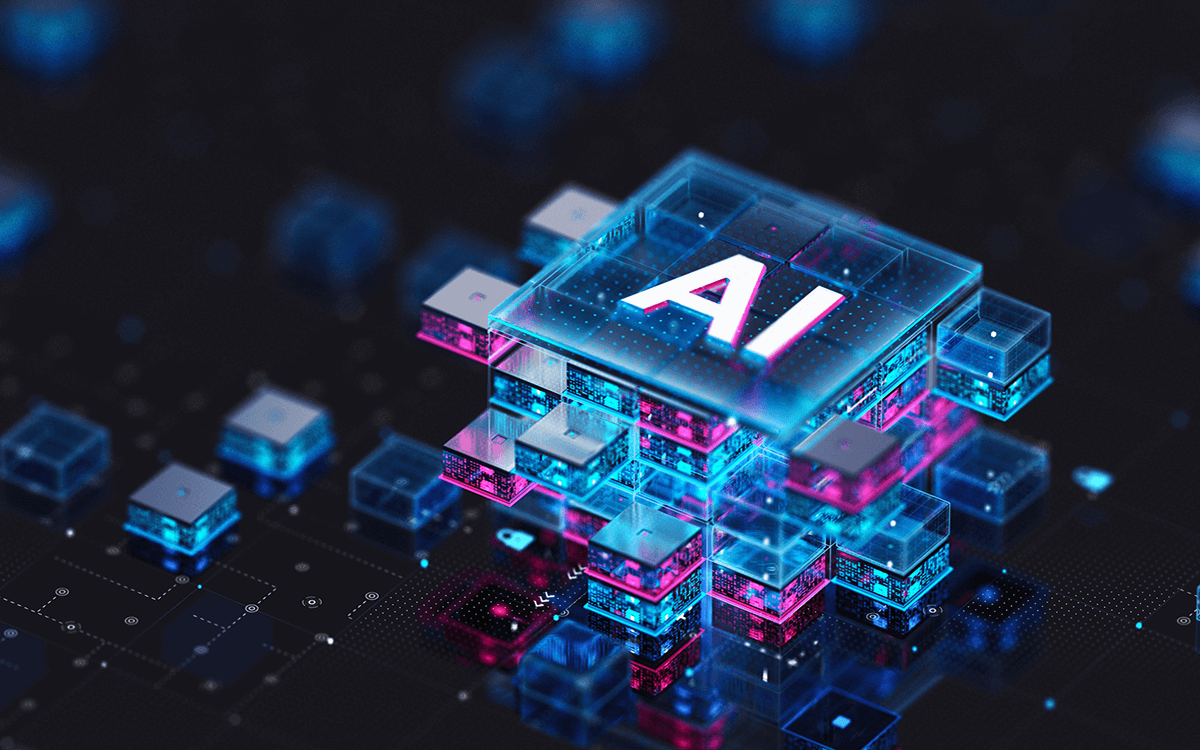
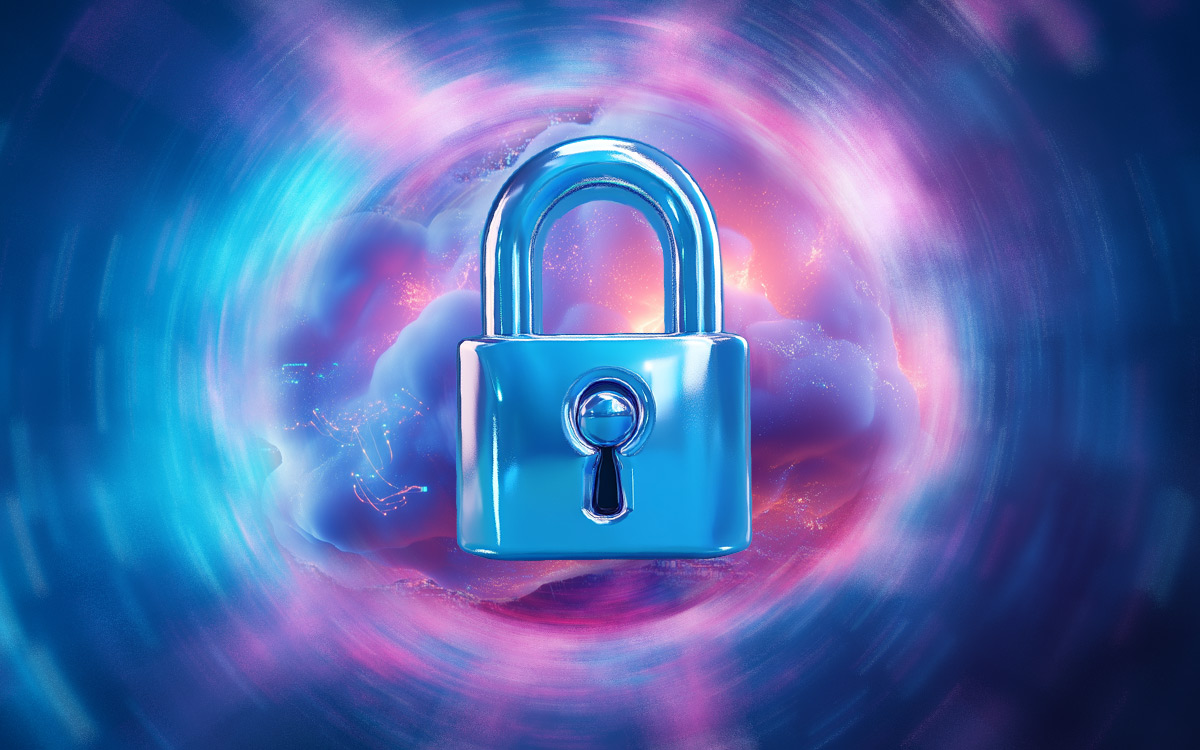
