Forefront of Banks' Digital Transformation: Generative AI Value Creation
Fujitsu / October 8, 2024
The banking industry, a mature market, differentiates itself through a few key competitive factors. To stay ahead and meet evolving customer needs, banks are increasingly adopting AI and other cutting-edge technologies. In recent years, generative AI has emerged as a transformative force with unprecedented potential to revolutionize various aspects of banking, from customer experience to risk management. According to a survey by the EIU think tank, 77% of banking executives believe that extracting value from AI is crucial for gaining a competitive edge. Therefore, leveraging the potential of generative AI for competitive advantage is a top priority for banking executives as they navigate the digital transformation landscape.
However, the current use of generative AI is largely confined to customer support functions, such as chatbots, and employee support, as well as some operational efficiencies. Its application to core operations remains in its infancy. In short, there is a significant gap between expectations and the actual adoption of generative AI. What is driving this gap? How can it be bridged? Banks need to harness generative AI to solve problems and create value that aligns with their strategic goals.
Contents
- Generative AI: Redefining the Role of Humans in Value Creation
- How to Use Generative AI in Banking
- Challenges Behind the Caution of Generative AI Adoption
- Fujitsu's Approach to Solving Issues in Adopting Generative AI and Maximizing Value Creation
- Conclusion: A Future Bank Intertwined by Humans and AI through AI Transformation
Generative AI: Redefining the Role of Humans in Value Creation
Generative AI, powered by large language models (LLMs), fosters a collaborative environment where humans and machines work together, significantly boosting bankers' productivity and enhancing human capabilities (see Figure 1).
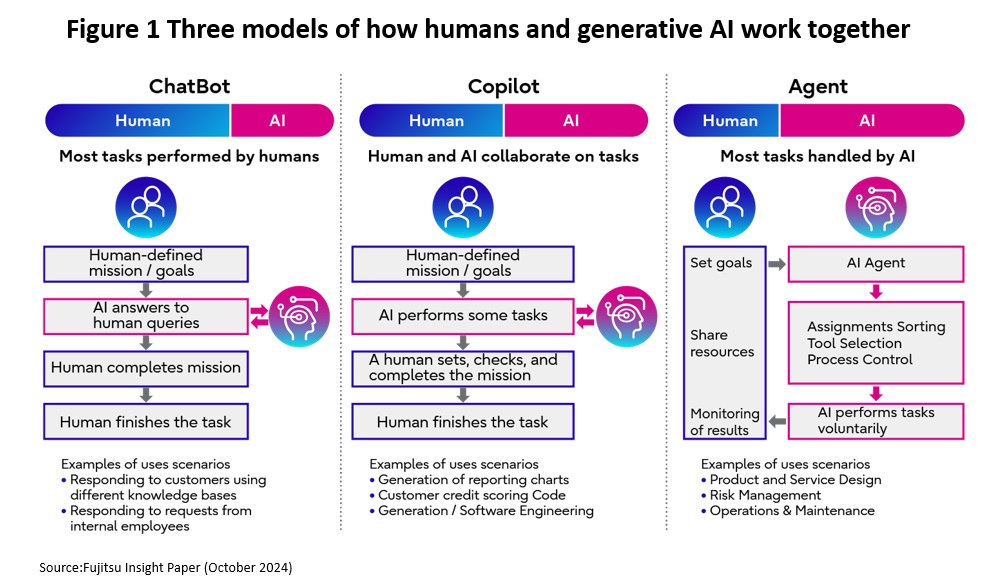
The banking industry is inherently language-centric, dealing with vast amounts of transaction data and human interactions. This makes it particularly well-suited for generative AI, which excels in processing and understanding language. For instance, generative AI can augment human capabilities across a wide range of roles, from back and middle office functions to front office tellers, wealth management advisors, relationship managers, call center agents, R&D staff, middle managers, and executives.
How Generative AI Can Be Used in Banking
There are four primary ways generative AI can be utilized in banking:
(1) Solution Enhancements
Integrate generative AI into commercial solutions or homegrown systems. Examples include Microsoft 365 and Salesforce's CRM assistant (Einstein). This integration can enhance productivity, efficiency, and employee satisfaction within the bank.
(2) Content Integration
Aggregate and analyze vast amounts of information to gain insights. This includes transcribing and summarizing customer call recordings, extracting information from research reports, leveraging external resources through RAGs (*1), anomaly detection, and customer sentiment analysis. These applications can improve productivity and strengthen risk management.
(3) Transform Software Development
Use generative AI to convert text and natural language into code across various programming languages. Code assistants, which use natural language prompts to update legacy code, are promising tools. This can enhance the productivity of banks and increase software developers' satisfaction.
(4) Differentiate Product and Customer Experience
Innovate and differentiate products, marketing strategies, and customer interactions using generative AI. Examples include developing personalized products and services, creating customer experiences informed by behavioral economics, and customizing pricing. These innovations can improve customer satisfaction, retention, and spending, significantly contributing to the bank's bottom line.
Generative AI holds the potential to dramatically enhance the efficiency of banking models. However, its most compelling potential lies in differentiating and driving growth by improving the customer experience. As shown in Figure 2, Accenture estimates that early adoption of generative AI over the next three years could increase productivity by 20% to 30%, boost revenue growth by approximately 6%, and raise return on equity by about 3%.
(*1) RAG (Retrieval Augmented Generation): A mechanism for acquiring and using knowledge outside of learning in LLM.
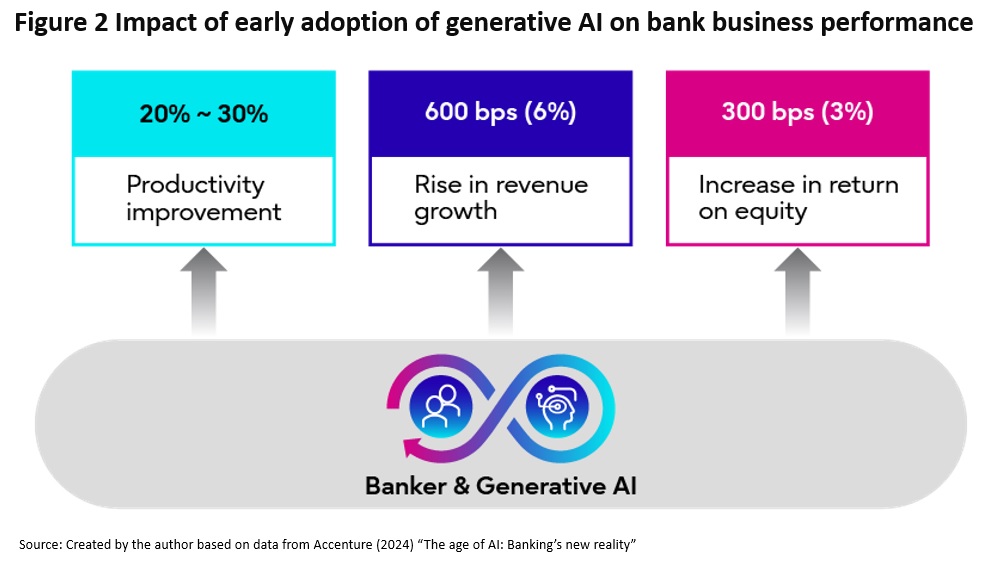
Challenges Behind the Cautious Adoption of Generative AI
Despite the significant business impact generative AI can have on bank management, its current use is largely confined to improving customer support and operational efficiency, such as chatbots and banker support, with its application to core operations still in its early stages.
Even in the United States, where generative AI technology development and ecosystems are highly active, the adoption of generative AI in the financial sector, including banking, remains cautious and modest (see Figure 3). In other words, there is a substantial gap between the potential use of generative AI and its actual implementation.
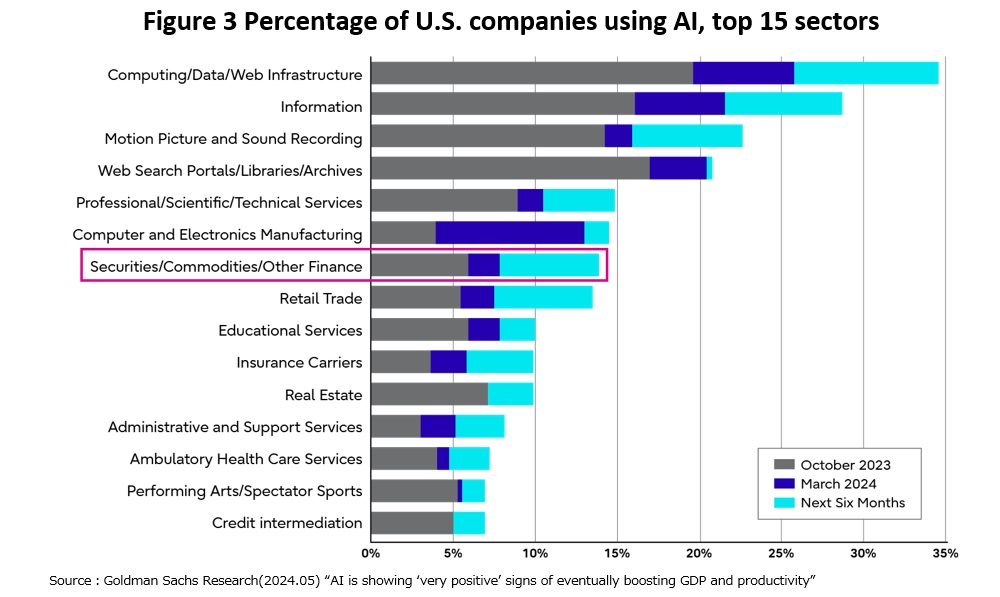
The author also examined the use of generative AI in several large banks (see Table 1). Overall, it is evident that each institution is proceeding cautiously, balancing the potential of generative AI with risk assessment. Most banks tend to start with low-risk, productivity-enhancing use cases (such as customer service, back-office operations, and coding) and gradually move to more complex applications as the technology evolves and matures, such as increasing revenue and enabling transformative potential for fraud prevention and front-office operations.
Despite being intrigued by the potential of generative AI, bank executives are reportedly cautious about adopting it for several reasons. There is growing awareness of the risks to customer data in generative AI, such as privacy breaches, toxic outputs (e.g., discriminatory content), intellectual property infringement, and poisoning risks (attacks via data and prompts). Additionally, compliance with evolving regulations and research into countermeasures to address these risks have been actively pursued, yielding successful results.
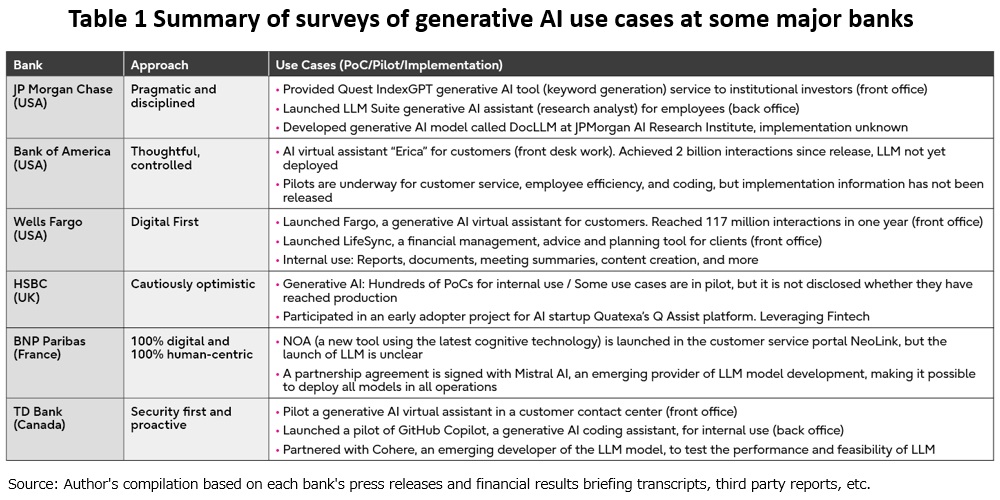
However, through a case study of a major bank, the author believes that the reasons for banks' cautious adoption of generative AI can be summarized in the following three points:
(1) Negative Impact on Competitiveness Due to Leakage of Proprietary and Confidential Data
Data owned by the bank is crucial for protecting customer privacy and serves as a key competitive differentiator. Banks are reluctant to use this data to train models, necessitating a way to leverage generative AI while safeguarding sensitive information.
(2) Tolerance for Errors (Such as Hallucinations) in Generative AI Outputs
The banking industry is built on trust relationships with customers, and face-to-face interactions have traditionally been important. The introduction of generative AI brings the risk of errors, such as hallucinations, which can lead to significant losses and erosion of trust. Therefore, caution is essential.
(3) Uncertainty in Value Creation Goals (Productivity Gains and Revenue Increases That Exceed Costs) Achieved Through Generative AI
As seen in the advanced case of a major bank, the use of generative AI has progressed from the learning phase of individual PoCs and pilots to the scale-up phase of implementing multiple use cases. However, turning the potential of generative AI into real business value is much more challenging than a PoC or pilot. It is not just about technical feasibility but about maximizing the value of generative AI through innovation.
Fujitsu's Approach to Solving Issues and Maximizing Value Creation in Generative AI Deployment
To fully realize the potential of generative AI in value creation, it is essential to address the issue of leakage of proprietary information (data) inherent in generative AI, significantly improve the phenomenon of hallucinations, and maximize profits (maximize return on investment) by scaling up (adopting multiple use cases) across the entire enterprise rather than for individual use cases.
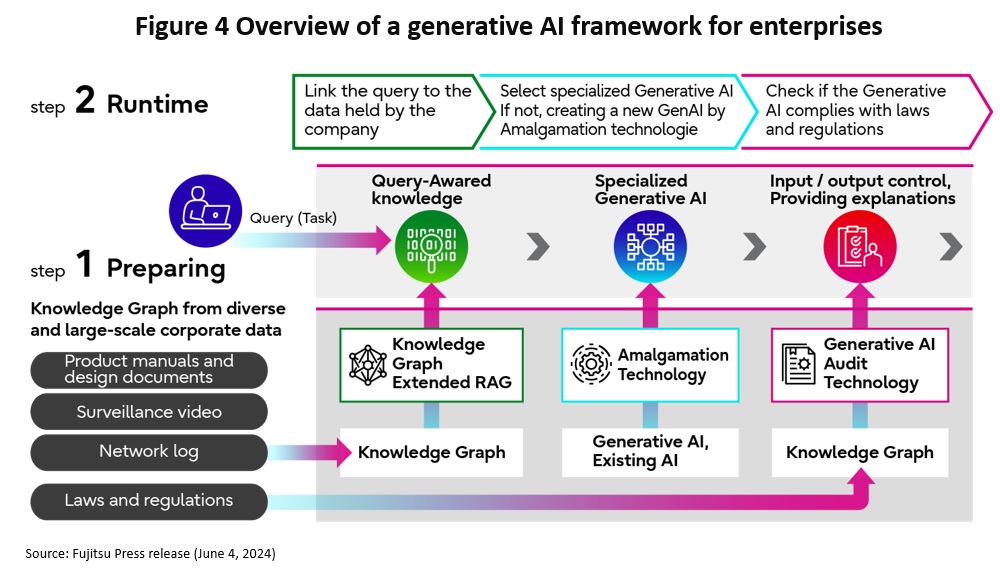
Aiming to be a leading global player in the enterprise use of generative AI, Fujitsu has launched the Enterprise Generative AI Framework globally (*2). This framework supports the entire enterprise (multiple use cases), addresses challenges in the use of generative AI within enterprises (including banks), eliminates security concerns, and delivers highly reliable results. As shown in Figure 4, it consists of three core technologies:
(1) Knowledge Graph Expansion RAG:
For diverse, large-scale enterprise data.
(2) Generative AI Amalgamation Technology:
Flexibly responds to changing enterprise needs by utilizing appropriate AI, including existing AI.
(3) Generative AI Audit Technology:
Behavioral control to mitigate the fear of using AI and ensure compliance with rules.
Generative AI then orchestrates these technologies to deliver reliable results.
Fujitsu's approach is unique in that it allows user companies to not only utilize a variety of general-purpose LLMs such as ChatGPT, Claude, and Gemini as their main tools but also to select or combine multiple models (including smaller and medium-sized models like Cohere and conventional models) that provide the highest performance for specific tasks. Additionally, it extends LLMs with systematic data in the form of knowledge graphs (*3) that organize knowledge such as organizational regulations.
For banks, implementing Fujitsu's Enterprise Generative AI Framework and synthesizing AI models they have already built is expected to improve asset and cost efficiency, enhance competitiveness through differentiation by leveraging their own data, improve output accuracy, and reduce or eliminate risks such as the leakage of confidential information.
(*2) Fujitsu Press Release (June 4, 2024)
(*3) Knowledge graph: A knowledge network that systematically represents various types of knowledge in a graph structure.
Conclusion: A Future Bank Interwoven by Humans and AI through AI Transformation
Overall, to address the challenge of expanding the adoption of generative AI, it is crucial to utilize external tools such as multi-modeling and RAG to automatically select the most appropriate model, thereby improving data security, output reliability, and efficiency. However, the adoption of generative AI also faces the challenge of increasing model development and operational costs. In particular, operational costs far outweigh development costs, and enterprise-wide model deployment can be expensive. Promoting the modularization and capitalization of common functions, as suggested by the author in a February 2024 Insight paper (*4), and building a unified platform for the entire enterprise can achieve rapid scale-up.
In the future, human-machine collaboration will shift to AI-driven business processes using LLMs, and human resources will transition to more sensitive and creative work, as well as roles such as monitoring and approval. AI transformation in banks will facilitate this human-AI collaboration.
For a detailed analysis of the use of generative AI in banking, please read the Fujitsu Insight paper: "Innovating Banking with Generated AI: Exploring Use Cases and Creating Value".
(*4) Jianmin Jin (February 2024) “Leveraging the LLM: Strategy from Model Selection to Optimization Insight for top management”
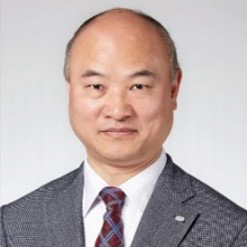
Editor's Picks
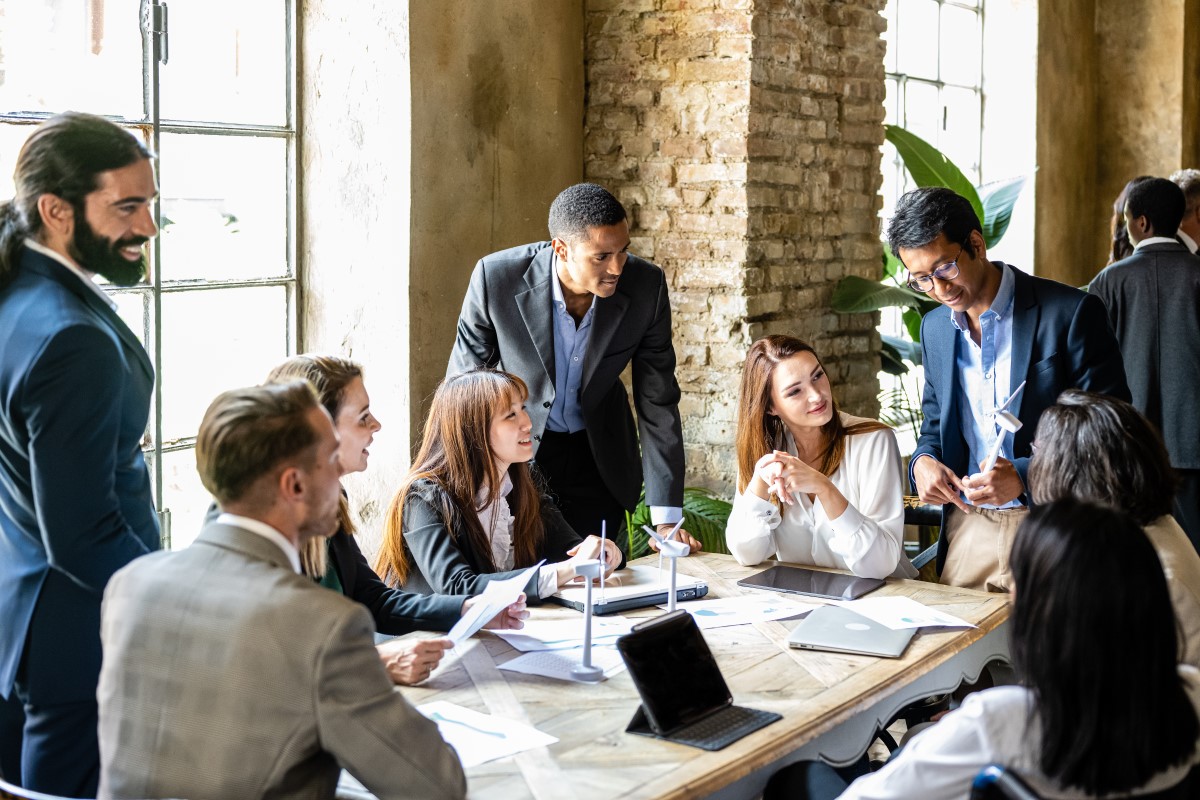
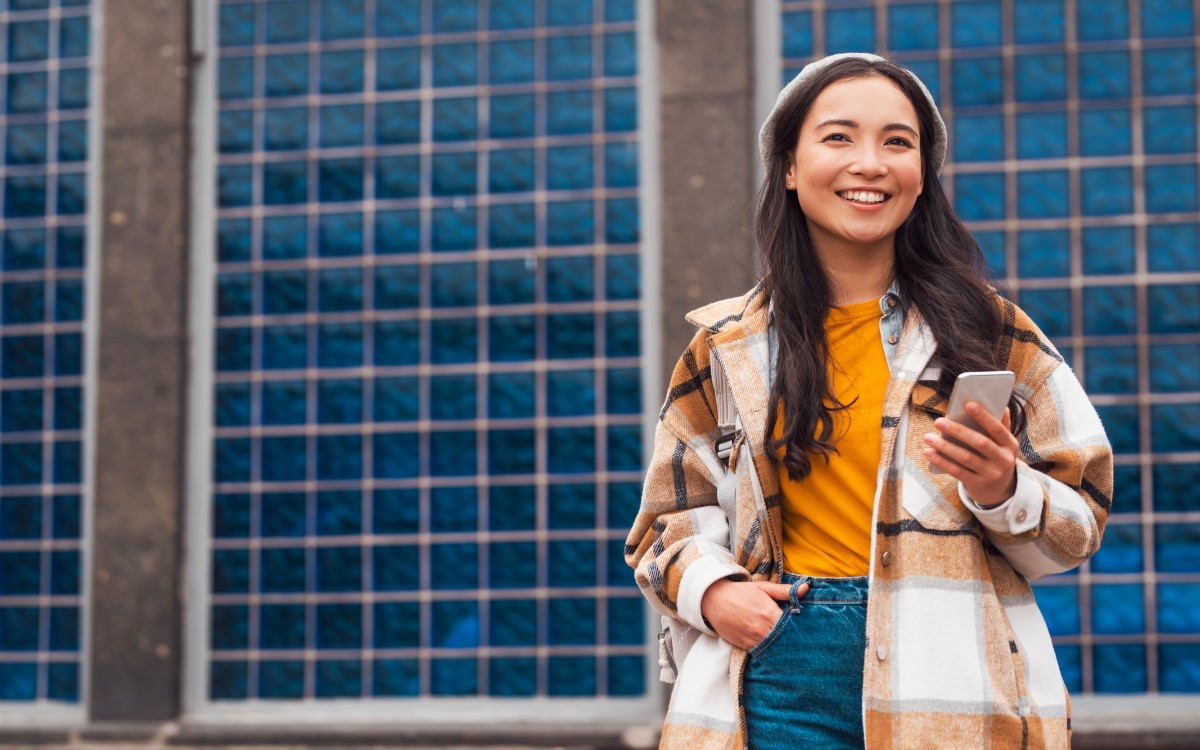