AI Model Collapse: Identifying and mitigating the risks
Fujitsu / September 19, 2024
AI model collapse is a newly recognized phenomenon of increasing concern to both AI developers and organizations leveraging the power of AI. The issue of AI model collapse was recently highlighted by Oxford and Cambridge Universities, published in Nature.
In a world where AI is increasingly intertwined with mission critical business processes, safeguarding against model collapse is not just a technical necessity but a strategic imperative.
Understanding AI Model Collapse and the developing risk
The research report by Oxford and Cambridge Universities, published in Nature, found that recursively training AI models on datasets containing AI-generated content can create a destructive feedback loop. This loop tends to favor AI-generated data over human or real-world data. Over time, this selection process amplifies errors, leading to increasingly distorted and hallucinatory outputs from the models.
The issue is especially pronounced for Large Language Models (LLMs) such as GPT-4o, which are trained on vast amounts of information scraped from the internet. Initially, this approach provided the diversity needed for robust AI models. However, the internet is now increasingly populated with AI-generated content. As this percentage grows, models trained on such AI-generated content will become increasingly susceptible to AI model collapse. However, this phenomenon is not limited to LLMs. Organizations that use AI-generated output as input for their own models recursively are also vulnerable to AI model collapse if appropriate steps are not taken to help mitigate the issue.
AI Model Collapse mitigation
The threat of AI model collapse requires that organizations adopt a proactive approach to mitigate future risks. Fortunately, two powerful AI technologies Retrieval Augmented Generation (RAG) and Graph AI when used appropriately together can offer a potential solution.
The role of RAG in mitigating model collapse
Retrieval-Augmented Generation (RAG) combines the strengths of retrieval-based systems and generative models. By integrating an external knowledge retrieval process into generative AI models, RAG overcomes many of the pitfalls associated with model collapse.
1. Ensuring Data Freshness: One of the core strengths of RAG is its ability to retrieve the latest information from a dynamic knowledge base, which can be continually updated. This directly combats data staleness, as the model is not solely reliant on its training data, but can pull in fresh, relevant information when making predictions or generating outputs. By tapping into external databases, RAG ensures that AI models remain relevant in a changing world.
2. Reducing Hallucinations: Traditional generative AI models, such as GPT, sometimes produce “hallucinations”—outputs that are syntactically correct but factually incorrect or irrelevant. RAG mitigates this by grounding generations in retrieved facts from a trusted knowledge base. The retrieval step introduces an additional layer of validation, reducing the risk of generating inaccurate or misleading information. This makes RAG particularly valuable in high-stakes environments such as healthcare, finance, and legal sectors, where hallucinations can lead to profound consequences.
3. Adaptive Learning Without Full Retraining: One of the key challenges with AI models is the need for frequent retraining to ensure they stay relevant. RAG offers a way around this by augmenting models with the ability to dynamically query external data sources. This reduces the frequency of retraining cycles, as the model can simply retrieve and generate answers based on up-to-date information, rather than relying solely on its pre-trained knowledge. As a result, organizations can extend the lifespan of their models without compromising performance.
4. Customization and Flexibility: RAG allows for more customizable and context-aware AI solutions. Rather than a one-size-fits-all approach, businesses can tailor the retrieval process to suit their specific needs, ensuring that the AI is drawing from the most relevant and trusted data sources for each query. This flexibility is key in maintaining trust in the AI’s outputs, especially in environments where accuracy and specificity are critical.
The role of Graph AI in mitigating model collapse
Graph AI leverages the power of graph-based data structures to model complex relationships and dependencies in data. This technology excels in understanding interconnected data points, which is essential for maintaining robustness in AI models and avoiding model collapse.
1. Mapping Data Relationships: One of the key drivers of model collapse is the failure to account for the interdependencies within data. Many traditional models treat data points as isolated units, which can lead to missed insights and erroneous predictions. Graph AI excels at mapping complex relationships between different data points, uncovering patterns that may not be immediately obvious in traditional data formats. By integrating Graph AI, models can gain a deeper understanding of the underlying structure of data, leading to more reliable outputs.
2. Real-Time Contextualization: Graph AI provides real-time insights into how data points relate to one another, offering contextualization that can prevent model collapse. For example, in financial services, a single transaction might seem innocuous, but when viewed in the context of a larger network of transactions, it may indicate fraudulent behavior. Graph AI can reveal these hidden relationships, allowing AI models to adapt and improve accuracy, even in changing environments.
3. Data Lineage and Provenance: Trust in AI models is often undermined by the “black box” nature of their decision-making processes. Graph AI helps address this by offering a transparent view of data lineage and provenance. By tracking how data flows through an organization and how different data points are interconnected, Graph AI ensures that the AI system’s decisions are based on a clear, traceable foundation. This transparency is crucial in maintaining trust in AI systems, especially when they are integrated into compliance-heavy industries such as healthcare and finance.
4. Detecting Shifts and Model Drift: Graph AI is particularly effective at identifying shifts in data patterns, helping detect when model drift might occur. For instance, in supply chain management, Graph AI can track changes in supplier relationships or disruptions in the flow of goods. By understanding these shifts in real time, businesses can adjust their AI models, accordingly, ensuring they remain accurate and trustworthy.
Synergy of RAG and Graph AI: A holistic approach to elevating AI model resilience
While RAG and Graph AI individually offer substantial benefits for addressing AI model collapse, their combination can create even greater resilience by enabling models to access the most relevant information and understand it within a broader context.
1. Contextual Retrieval: Graph AI can enhance RAG’s retrieval process by guiding the system to retrieve the most contextually relevant information. By understanding the relationships between different data points, Graph AI helps narrow down the retrieval process, ensuring that the data fed into the generative model is not only up to date but also contextually appropriate. This results in more accurate and reliable outputs.
2. Explainability and Trust: Both RAG and Graph AI contribute to explainability RAG by linking outputs to specific retrieved sources and Graph AI by mapping the relationships that underpin the data. Together, they create a robust framework for explainable AI, where users can trace back decisions to specific data points and understand how those data points relate to one another. This enhanced transparency is critical for maintaining trust in AI systems, particularly as they are deployed in high-stakes environments.
3. Dynamic Adaptation: Combining RAG and Graph AI allows AI models to dynamically adapt to changing conditions without requiring constant retraining. RAG ensures that the model has access to the latest data, while Graph AI tracks evolving relationships between data points. This dynamic approach enables AI systems to remain robust in the face of evolving patterns, reducing the risk of model collapse.
4. Scalability Across Domains: The combination of RAG and Graph AI is applicable across a wide range of industries and use cases. In e-commerce, it can improve recommendation engines by retrieving up-to-date product information and understanding customer preferences in a networked context. In healthcare, it can enhance clinical decision support systems by retrieving the latest medical research and understanding patient histories in relation to broader treatment patterns. This scalability ensures that businesses in diverse industries can leverage the combined power of RAG and Graph AI to safeguard their AI systems from collapse.
Conclusions
As AI becomes a cornerstone of business strategy, the risk of AI model collapse presents a significant challenge. Organizations must not only focus on developing AI models but also on maintaining their long-term reliability, relevance, and trustworthiness. Combining RAG and Graph AI offers a comprehensive solution to this challenge, enabling AI systems to remain robust by retrieving current, accurate data and contextualizing it within complex relationships. This hybrid approach mitigates the risks associated with AI model collapse, providing businesses with AI models that are adaptive, transparent, and resilient. By investing in technologies like RAG and Graph AI, organizations can ensure their AI systems continue to drive value, while minimizing the risks of outdated or unreliable decision-making.
So, why not talk to Fujitsu and find out how we can help your organization harness the power of RAG and Graph AI to improve the resilience of your AI models.
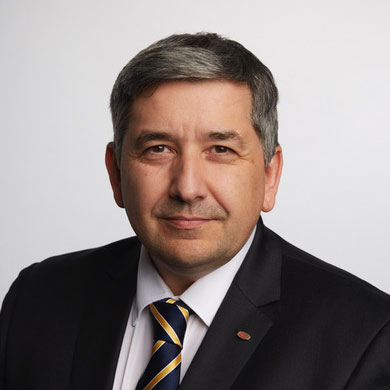
Related information
Editor's Picks
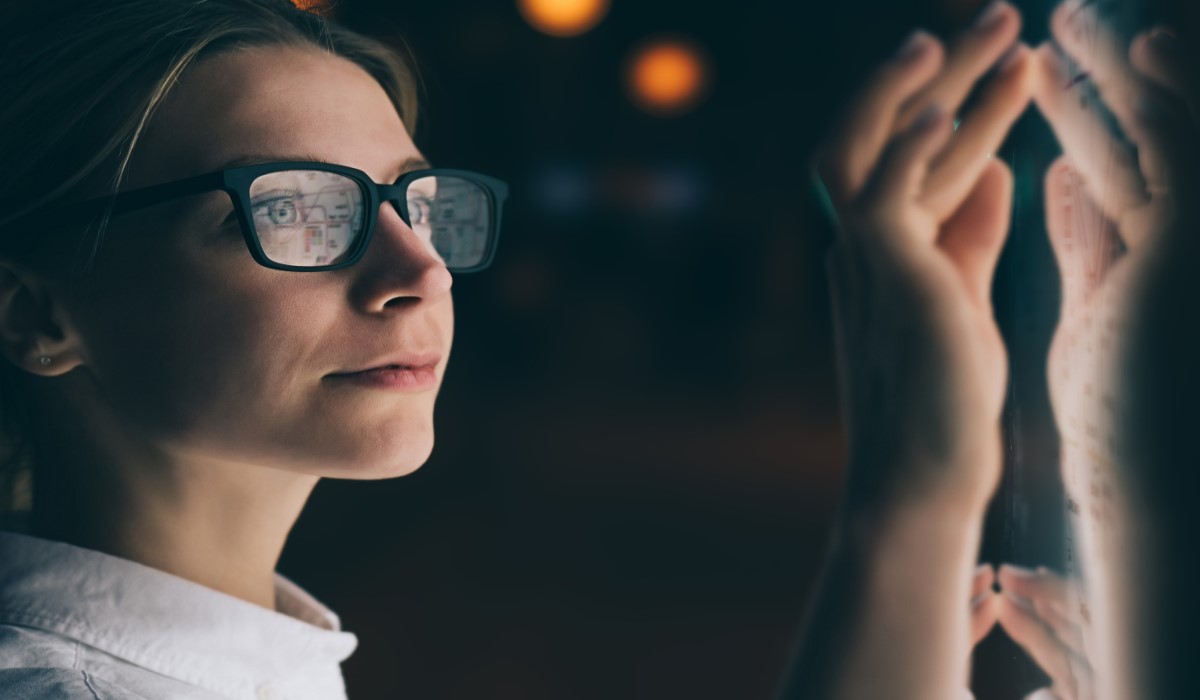
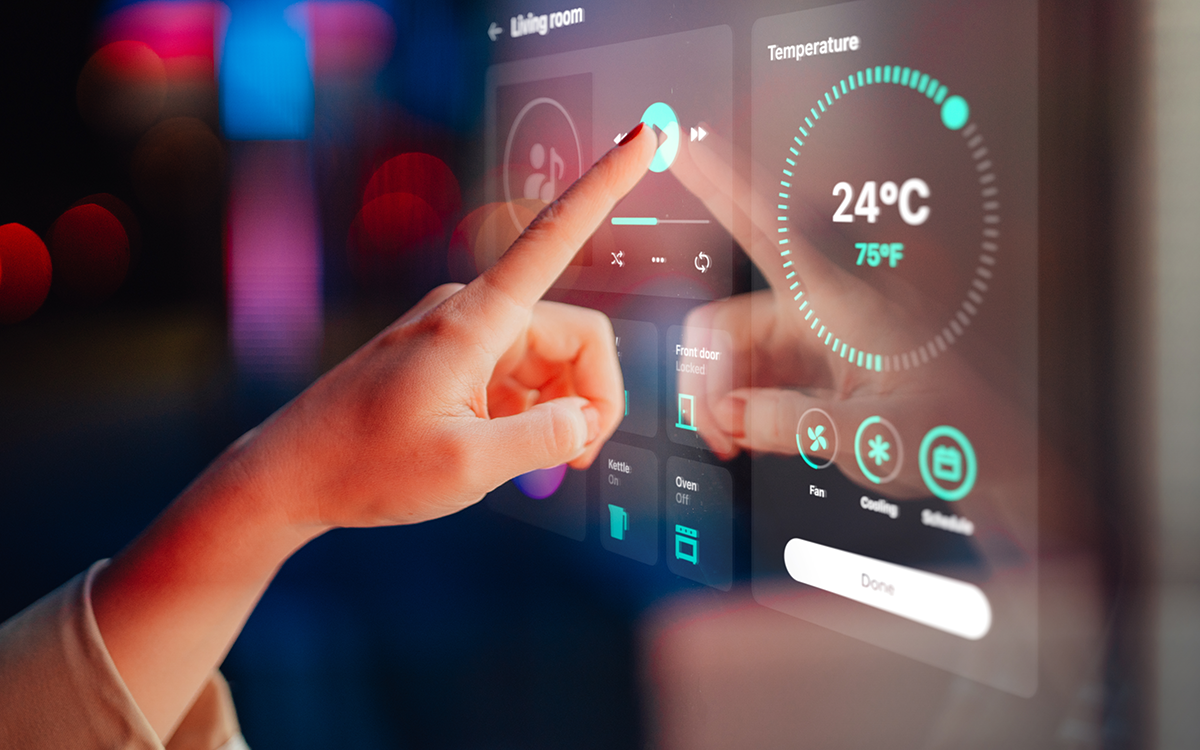
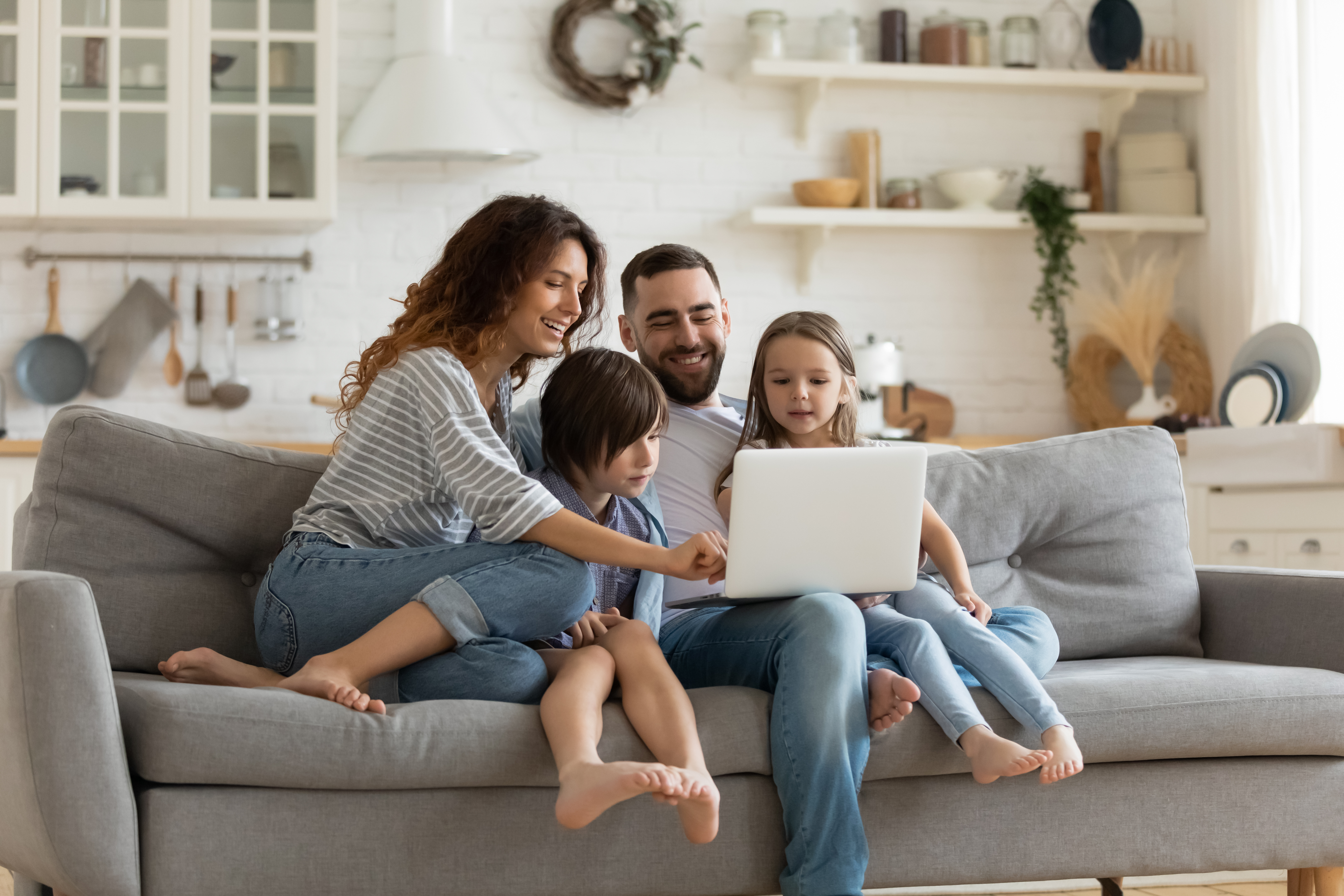