Cutting through the AI hype: A pragmatic guide for CXOs to deal with exponential technological change
Fujitsu / March 1, 2024
The implicit promise of AI - that it will be the first generation of automation that adapts to humans, rather than humans having to adapt to it - is a fallacy, says Neil Lawrence, Deep Mind chair at Cambridge University. (*1) Every other new technological revolution changed how processes occur, businesses are run, and how society is organized. There is no evidence that AI is different. But the AI fallacy continues to distort how organizations think about AI. Many leaders start from the assumption that AI can be added as an easy bolt-on for dramatic business transformation.
I have had the privilege of engaging in discussions about the implementation of AI as a solution to various social and organizational challenges. In this article, I aim to share insights and practical advice from those discussions for CXOs.
The ‘one thing’ illusion of AI
Common parlance reveals a prevalent misconception that many people may not even realize they hold—that AI is a single thing. It is often not recognized that the field of AI encompasses a vast array of technologies and tools, each with unique capabilities and applications. For instance, while neural-inspired AI systems excel at pattern recognition and learning, rules-based systems provide clarity and explicability where decision-making processes need transparency. Drug discovery, for example, requires a fundamentally different set of AI tools compared to face recognition. They operate in distinct ways.
The challenge for business leaders is that indicating “we will use AI to improve” is simply not sufficient. Success is only viable by identifying the technology that aligns with their unique business challenges.
Clarity of business purpose
If a customer reaches out saying, “we would like to look into adopting AI.” I invariably respond with a polite version of “to do what exactly?” If they do not have clarity about how adopting AI will confer a business benefit, I expect that the conversation is unlikely to go anywhere. Unless the organization understands what the actual problems or opportunities are, technologists – theirs or ours – have a huge challenge to advise which of the myriad AI tools or components can be applied to derive that desired benefit.
A shorthand label for this sort of thinking, ‘the underpants gnome problem’, comes from a story in an episode of South Park. The lads find that their underpants are going missing. They track down a gnome stealing the underpants, and follow it down a tunnel to discover a vast pile of stolen underpants. At which point they ask the gnomes the most obvious question – “Why are you doing this?”
The gnomes respond with their simple three step plan.
“Phase 1 – Collect underpants.
Phase 2 – Uhmmm.. let’s put a pin on it for now.
Phase 3 – Profit!”
Of course, there is no Phase-2. Just a huge unfounded assumption that something will connect phases one and three.
This is why we sometimes have co-creation workshops where we brainstorm with key leaders from the customer’s organization and experts from Fujitsu; understanding what the key challenges are, before getting into solutions. We need forethought, clarity and should avoid the trap of thinking that AI will magically make things better.
The people aspect of an AI Strategy
AI never exists in isolation. AI tools will always be part of a wider system. AI is always interacting with people somewhere. For example, Large Language Model (LLM) outputs often act as prompts to human thinking as much as humans provide the prompts into LLMs. But understanding how the technology is best applied to individuals, teams, or organizations differs.
Empowering an individual isn’t the same as empowering a team. Let’s say an AI lets someone write more emails. If those are internal emails rather than mass marketing, it probably wouldn’t make the team more effective. Especially if their ability to respond to e-mails hasn’t improved. Supercharging the quality of the output of the individual, rather than just the volume makes more sense in that setting.
Aiding individuals will be helpful, but is unlikely – alone – to revolutionise the performance of their team. There is a good example from Wharton Business School, a team that builds educational tools. Incorporating AI to give feedback about the tool prototype saved the bandwidth of testers. Using AI to act as a live participant in a meeting, to take notes, collect human feedback, and make code changes all saved time. But it was only by changing workflows and patterns of behavior across the whole team, weaving AI into those systems, were they able to cut delivery times from over two weeks down to days. The astute reader here will already have seen the cost and potential source of resistance to overcome. Change like this is far more disruptive than simply giving a piece of AI software to an individual. There might have to be a change in how the entire team works with the introduction of AI.
The final level is the ‘organization’; this is quite distinct from the ‘team’. A team is small and connected enough that any one member can have tacit knowledge about all the other people in the team. Who they all are, what they are doing, who needs or can offer help. An organization is simply too big for that. It would be impossible for any one individual to know what everyone in HSBC or Google did for example. Organizational changes are either simple additions like the addition of a new software package all employees might use, like empowering the individual but at scale, or they can be large structural changes on how the business functions.
To understand the link between disruption and productivity, an example I use comes from the era of electrification.
Before electrification, many factories’ tools were belt-driven. The belts transferred mechanical power from overhead transmission rods running across the factory driven by some central engine. When electrification first happened, there wasn’t an immediate productivity boom. At least not a big one. Power was only required when the tool needed to run, overhead transmission rods weren’t required to turn continuously; but this was a small energy saving. The real change happened when the layouts of the factories themselves were changed. Production processes could be completely refined. In the belt driven era, the factory layout was governed by the efficiency of the rod-belt power lay out; not the ergonomics of activity required to make the product. The assembly line as we recognize it now was essentially impossible. Once the tools could be placed anywhere, they could be set out to enable a vastly more efficient and effective assembly process of people and machines. How the organization flowed had to change to realize the benefit, not simply swapping in substitute technologies into the existing business layout.
Understanding individual behavior, team behaviors and processes, and organizational workflows, is fundamental to ensuring the success of AI deployment.
The ‘stopping problem’ of data quality
Any conversation about AI will be incomplete without giving a thought to data. The quality of the output from the AI system is often directly proportional to the quality of the data available. While this is true, one of things to be wary of is ‘a stopping problem.’ Some customers refuse to consider AI until they have perfectly prepared their data. The problem is that it may be impossible beforehand to know how good is good enough. Some additional investment of effort might increase data quality. But each increment of effort typically confers a little less gain. Never knowing when to stop with slowly diminishing returns but never quite sure if sufficiency has been reached. Sometimes you just need to pick a point to start and accept that data cleaning must mature through the application phase over time.
An example of a more productive iterative mindset is described by AI pioneer Andrew Ng about students of his who founded a start-up. They began by building a dataset of photographs of diseased and healthy cabbage. Then they created a computer vision system to identify diseased cabbage to target and eliminate the pest threat, and thus cut down on the blanket usage of pesticides. With their prototype system, they went to farmers who found their system useful. But that initial set of users generated many more photographs, and thus more data to build an even better AI system. Which in turn resulted in even more users. Ultimately, the agricultural manufacturing behemoth John Deere, snapped up Blue River for over $300 Million, because the dataset was so unique and difficult for competitors to obtain.
Some people say that data is the new oil. But oil is extracted; data is made. Oil is consumed when used; data can be reused repeatedly. The right mindset often isn’t one of finding the cut off in the ‘stopping problem’ but to think about building cycles that lead to iterative improvement.
The right time to adopt AI
Another question that I get asked is whether it is the right time to adopt AI. Should they be early adopters of an emerging technology or wait for it to mature before committing to use it?
This is not a simple problem. Humans are bad at intuitively grasping the realities of exponential growth. Furthermore, overall exponential general technology growth is composed of steps of individual transformative technologies; where each step typically grows in a sigmoid, S-shaped curve. Initially, there is slow progress; often accompanied by over-promising and disappointment. The middle phase is where it explodes into growth. Then, in the final phase, progress levels off; the technology is essentially mature and only the most challenging applications remain.
Vaccinations serve as a great example. The concept of vaccinations has been around since at least the 15th century. Initially, there was a slow and gradual increase in understanding as people tried to determine the amounts and types of diseases that could be given to someone to induce immunity without actually causing the disease. Once the foundations were well understood there was a suddenly acceleration of vaccination successes particularly through the 60s and 70s. As we manage to vaccinate against more and more diseases, we'll be left with only the most challenging cases, and progress will plateau.
Right now, the greatest excitement in AI is about Gen AI and LLMs, because that is the current steepest curve. The assumption is that this part of the curve will solve most future problems and should be the tool of choice. But this belies the understanding where each of the different AI tools is on the broader AI S-curve, and which combination is the right fit for each specific business problem.
In some cases, it may not be possible to understand which is the right collection of tools and approaches without experimentation. But such experimentation demands time, money, and business disruption; it is a cost. But if you cannot know in advance when the right moment is, then there are only two ways to react to exponential technological change: too soon... or too late. Frequently the move to adopt AI, especially in the face of some uncertainty, will feel too early. But if you don't or cannot understand when the right moment to adopt AI is, too early is more likely to be survivable than too late.
Conclusion
To enhance the likelihood of your AI project's success, it's crucial to understand AI as a toolkit rather than the ‘one thing’ illusion of a single fix-all magic wand. Clear objectives should guide your AI initiatives. The human aspect of AI should be considered from the outset and throughout the project. Starting with a reasonable level of data quality is important, but remember that improvement – like the AI cabbage farmers – is often best as an iterative process. Lastly, it will rarely feel like the ‘perfect’ time to adopt AI; but don’t wait until it is too late.
The best first step might just be to talk to us.
In the interim, if you want to explore an AI toolkit, feel free to look at Fujitsu’s ‘Kozuchi’; a cloud-based platform structured around 7 areas of AI, enabling rapid development, testing, and implementation.
www.fujitsu.com/global/kozuchi.
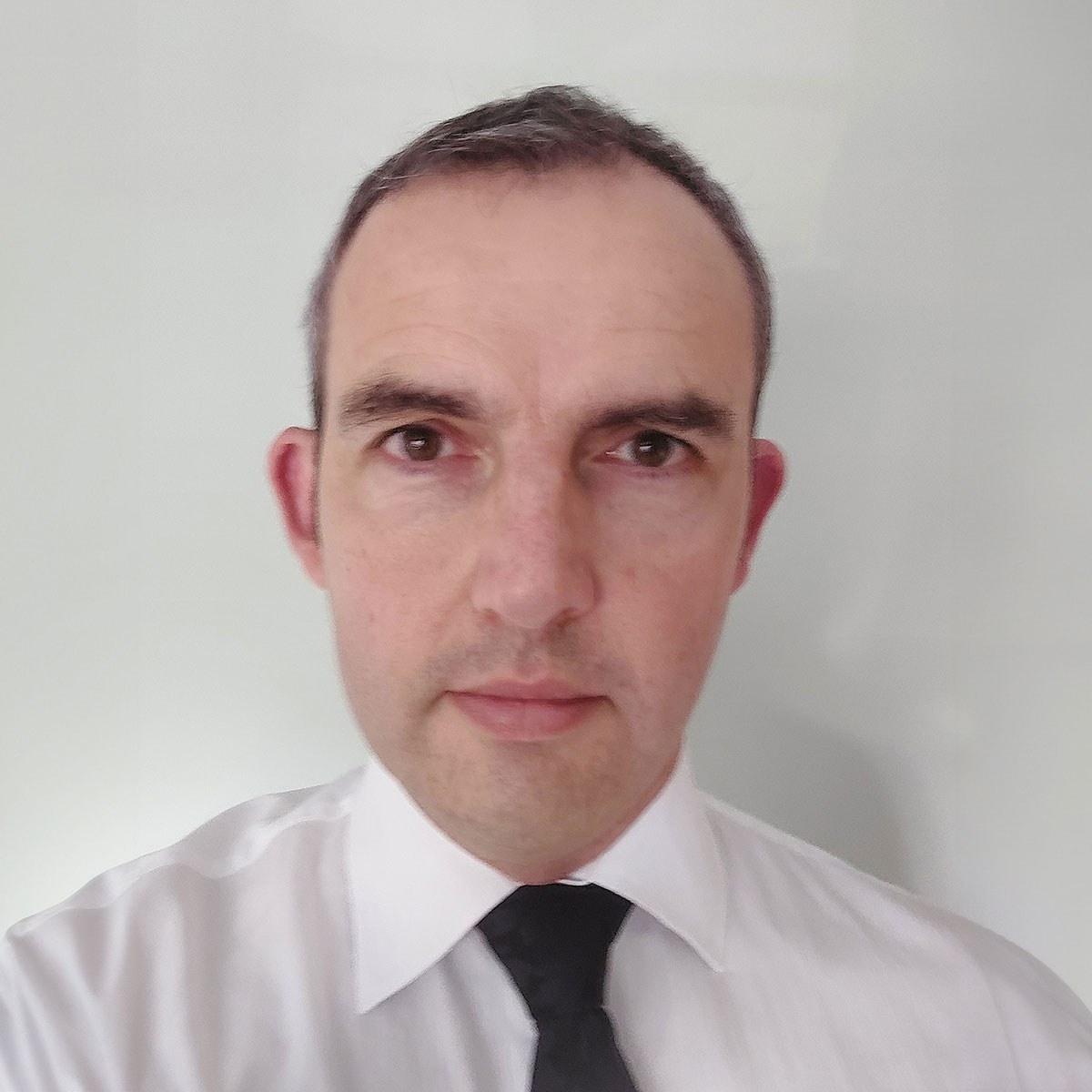
Al is a former Research Fellow at Oxford University and was the Chief of the General Staffs’ Scholar. His research at Oxford was on perception, information integration, and optimisation for competitive advantage in biological and computational systems. Al writes and has lectured on artificial intelligence, robotics, human-machine teams, and related subjects at the Alan Turing Institute, Oxford University, UCL, Cranfield University and other academic, industrial, financial and government institutions. He has also been one of the Group of Government Experts on Autonomous Systems providing advice to and speaking at the United Nations.
Al is also a former Army Officer, where, in addition to other operational tours, he commanded an Explosive Ordinance Disposal Regiment and led the Counter-IED Squadron in Afghanistan.
Related information
Editor's Picks
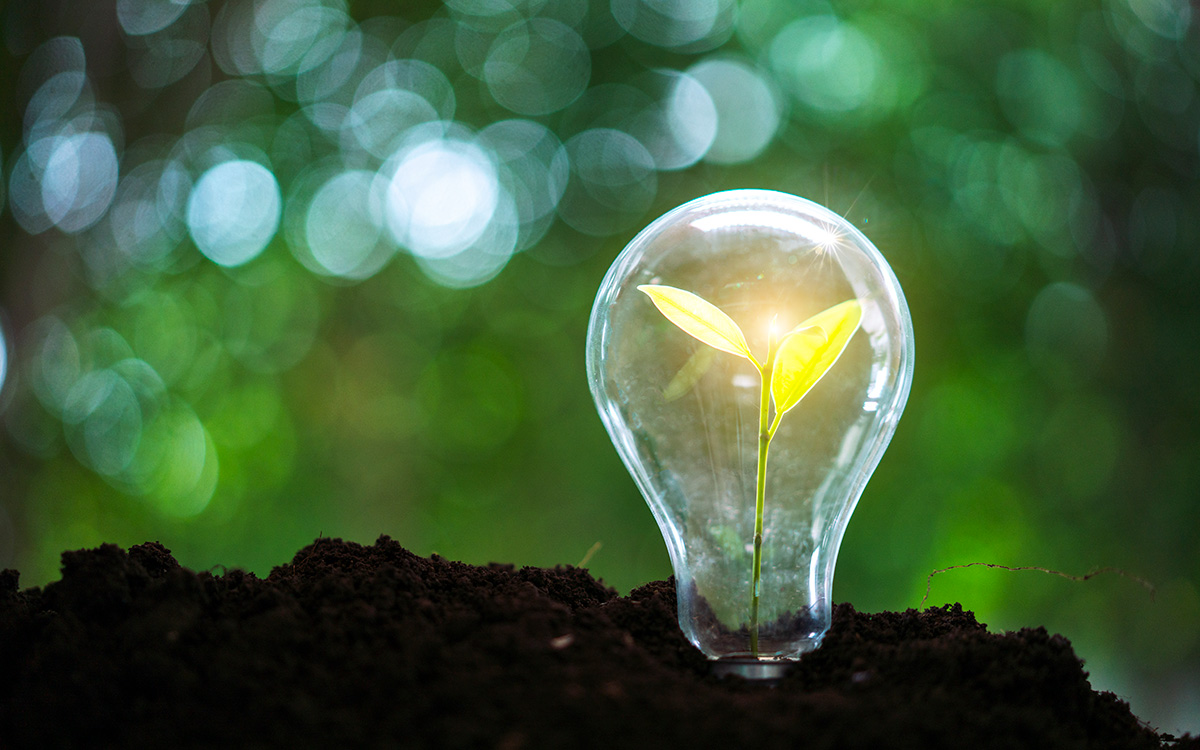
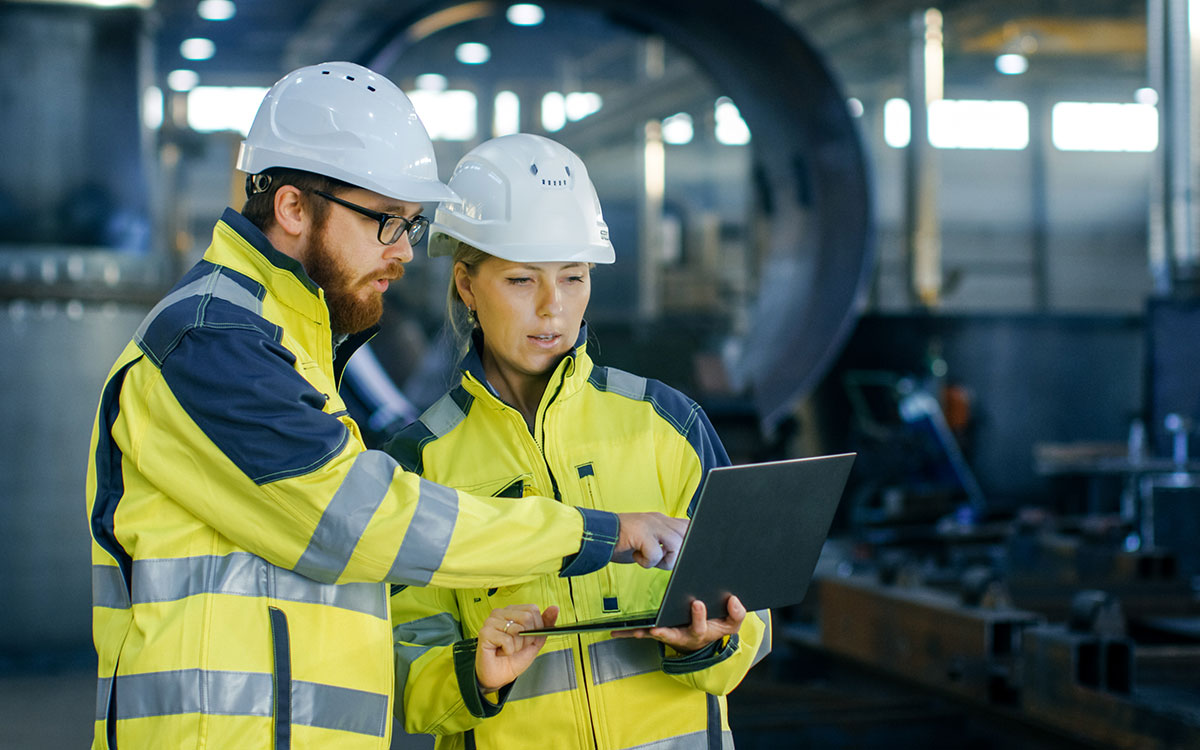
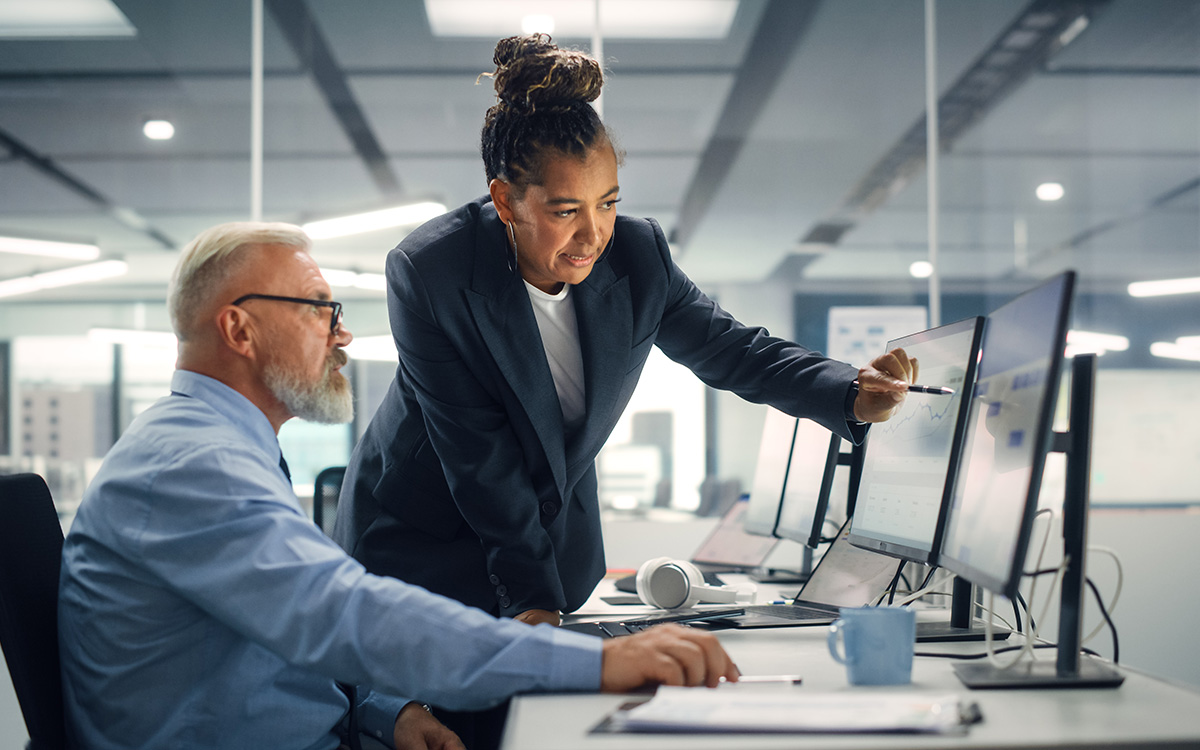