AI is already being used to help realize sustainable digital factories
Fujitsu / February 15, 2024
AI may have arrived just in time to help manufacturers facing the twin challenges of an aging workforce and aging OT, argues Jouko Koskinen, Senior Director, Global Digital Factory Offering.
Introduction
In Finland, we have an expression – “silent knowledge” – that means all the things that are so obvious to you where you work that nobody has ever bothered to write them down, let alone systematize them.
In factories, there tends to be a lot of silent knowledge. Manufacturing operations often need a few tweaks that people on the lines find out about over time. That knowledge gets passed from operator to operator and shift to shift, so things work.
Or it would be better to say there used to be a lot of silent knowledge. It was all in the heads of a generation now passing through retirement. As they disappear from payrolls, many factory management teams struggle to recruit younger factory workers willing to take up the same roles.
You might think me unduly optimistic, but maybe this is an opportunity.
The end of the line
First, the manufacturing industry is under extreme pressure to adopt new, sustainable production methods that reduce resource consumption and cut CO2 emissions. Circularity demands an entirely new way of thinking about manufacturing purposes – or, indeed, the purpose of manufacturing.
Second, the generation of automated production technology under the umbrella term “OT” is also nearing the end of its useful life. Patching up today’s OT (which most factories can’t consider just scrapping) to work with modern IT systems requires a new way of thinking.
Looking through these two prisms, a change in workforce generation may be quite helpful in fostering new ways of thinking.
AI in the factory
When it comes to the future of sustainable manufacturing, the technology causing the most interest is obviously AI.
Generative AI is getting all the attention right now, but it is best seen as the end-user interface. For actual productivity improvements you also need things like computer vision AI and AI models for specific tasks.
With AI, factories can locate precise and perhaps unexpected ways to reduce consumption across a broad range of measures. That’s not happening so much today because factory data monitoring tends to be siloed by department, line or machine. There is little in the way of holistic analysis of all the available sensor data to build a bigger picture that could lead to improved energy efficiency, reduced waste, and increased production output.
As AI starts to arrive in software vendors’ offerings, there is a risk that the same siloing gets replicated. Yes, each vendor is innovating, but within their own domain systems — MES, IoT, ERP, for example. Under these circumstances, manufacturing CIOs should be aiming for an integrated AI UX across multiple AI-enabled systems that access multiple factory data sources, and assisted by an AI partner with holistic thinking and long industry expertise.
With this cross-factory view in place, a manager would be able to talk or key a query to the interface, such as: “Suggest the three most effective ways we could reduce raw material usage in our factory in Poland.” The AI then queries multiple systems, including Accounts Payable, to discover what materials are being ordered; the MES to identify the number of raw materials being used, the number of goods produced and any incidents of downtime; Quality Control to check what is being rejected and at what stage; the WMS to find out what actually arrives into stock and what gets lost or damaged before it’s dispatched to customers; Accounts Received to check what is getting paid for; HR to unearth any labor shortages and underlying causes such as retirements or specific skills gaps — and so on.
Suggestions from the AI might point towards persistent overordering of raw materials due to poor quality of incoming materials, compounded by a growing percentage of specific parts rejected by QC. It might uncover an emerging issue with a particular machine or shift where the number of rejects is higher than expected. This might point to labor shortages – perhaps in skilled engineers identifying and servicing the defective machine or inexperienced operatives for specific processes, resulting in agency staff handling work for which they are not adequately trained. It might also identify issues in the warehouse, where the amount of stock being written off is on a negative trend, and a worrying imbalance on a narrow range of parts between the amount of raw materials bought and money received for the finished goods.
AI computer vision
Another way AI is opening doors for manufacturers is by using video from the many cameras that are probably already in their factories.
By analyzing camera data, AI can identify where processes are not being performed properly or safely. Computer Vision and AI can recognize what kind of work is being done, such as sorting, packing, and grinding, from a factory’s camera or video footage. This makes assessing and improving work processes possible, ensuring greater factory safety and eliminating difficulties, inefficiencies, and differences between workers.
Applying AI computer vision to automate QC is another use case for video, where it can cut down on raw materials wastage. Fujitsu has recently tested AI computer vision for a high-volume producer of metal ingots. It checks for surface defects as minor as 1mm2 during live production on materials that are too large and too numerous to expect human operators to be able to function efficiently and continuously. The system helps the manufacturer identify trends and patterns regarding when and where faults develop. The business impact of these insights is the ability to introduce targeted and effective interventions, resulting in fewer customer complaints, fewer compensation payments, heightened customer satisfaction, and an increasing brand reputation for quality.
How to get started?
The number of potential upsides from AI for manufacturers is probably limited only by the imagination to think of novel use cases. And gen AI can even help with that.
Maybe reading this, you have thought of how AI could achieve something really useful in your manufacturing setup. Reach out to us to have a conversation.
Incidentally, Fujitsu's proprietary cloud-based AI platform, called Fujitsu Kozuchi, focuses on seven areas of AI (Generative AI, AutoML, Predictive Analytics, Vision AI, Text AI, AI Trust, XAI) that can be rapidly developed, tested, and implemented to deliver immediate results.
To learn more about how Fujitsu Kozuchi can help your organization solve social and organizational challenges using AI, visit: www.fujitsu.com/global/kozuchi.
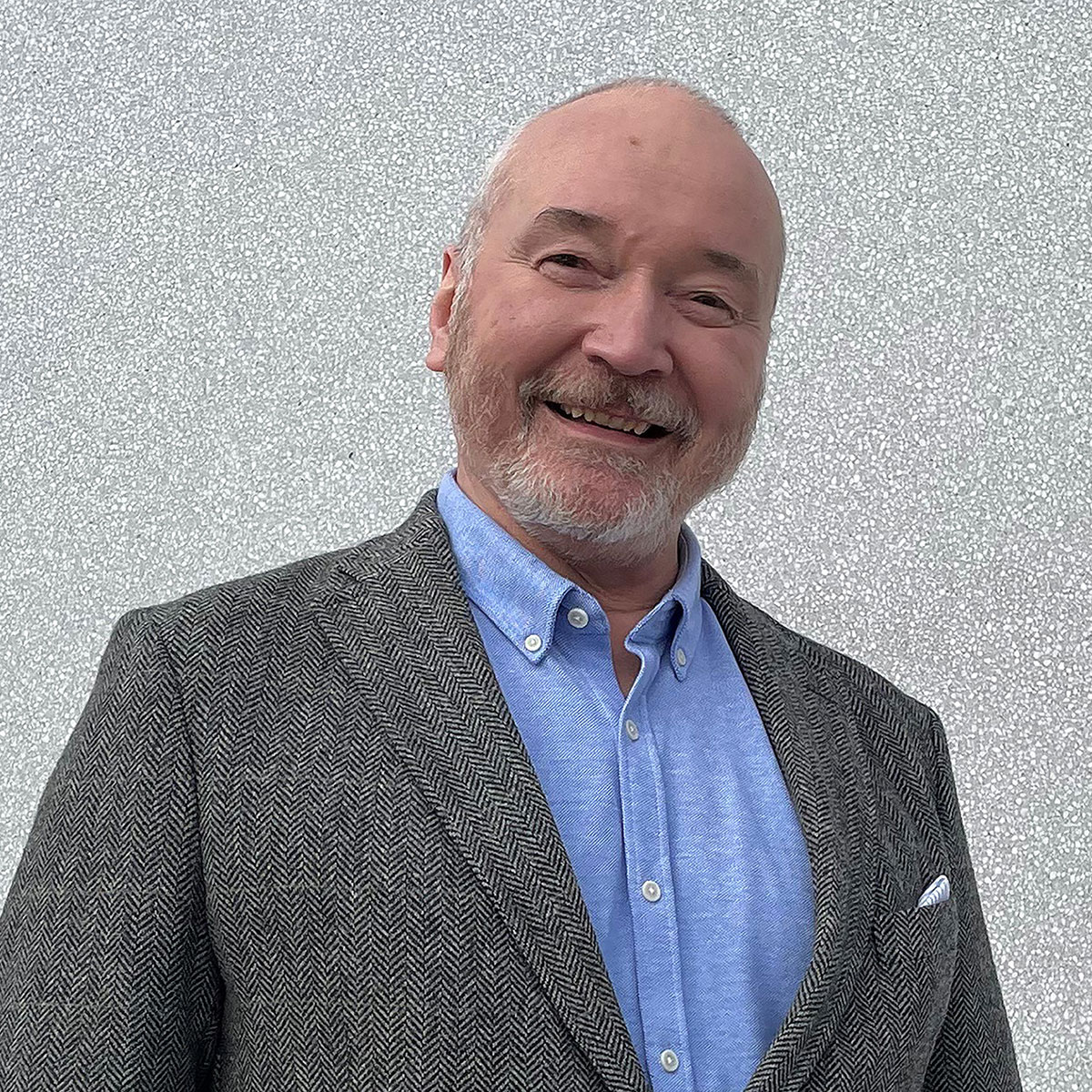
Related information
Editor's Picks
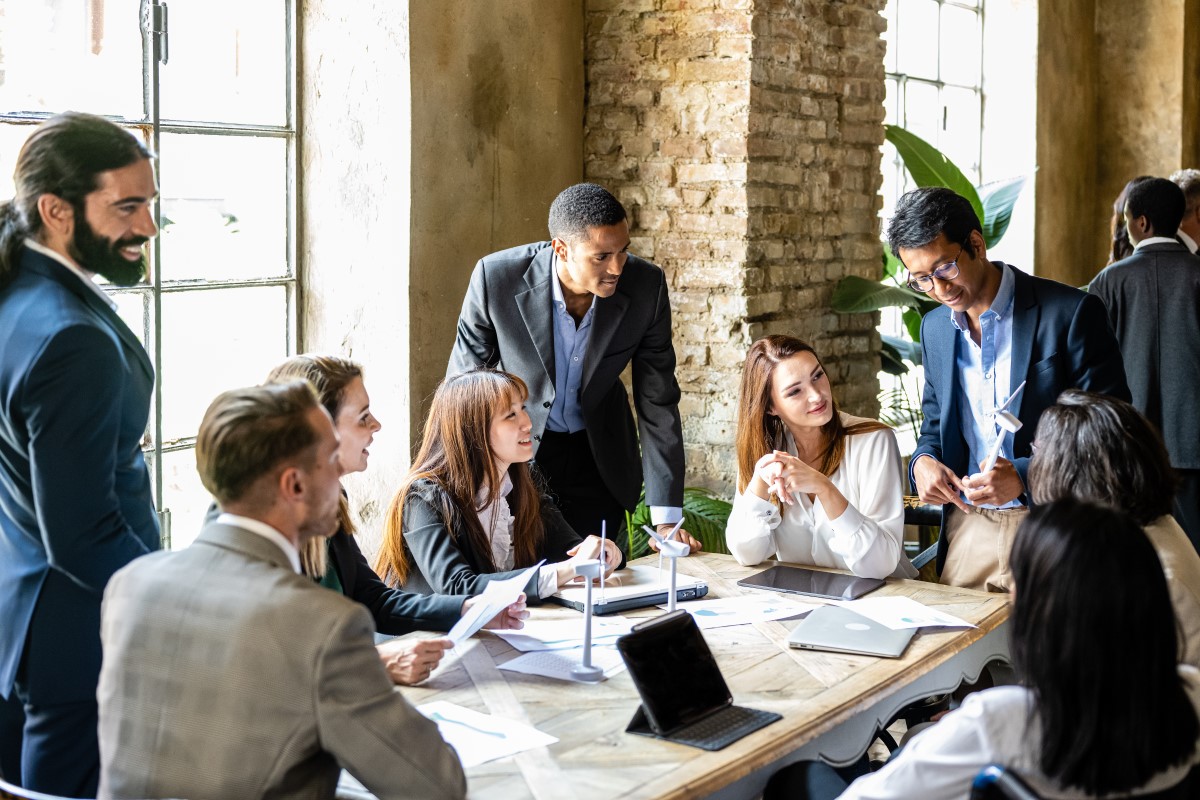
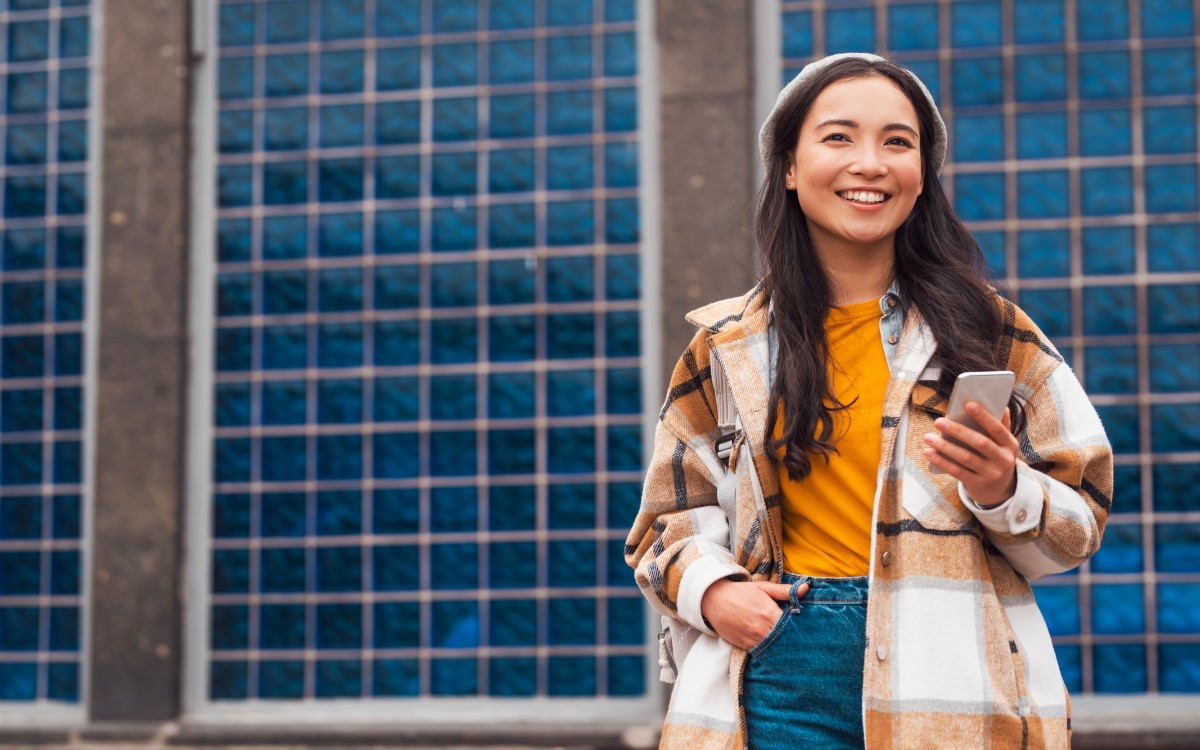