Revolutionizing productivity with Generative AI: a new epoch of value creation
Fujitsu / January 19, 2024
ChatGPT, along with other similar applications, has showcased the immense potential of generative AI in value creation. It provides an innovative platform for engaging in human-like dialogues, generating fresh content, and revolutionizing work processes, thereby enhancing productivity and facilitating insightful exploration of the future. Indeed, the adoption rate of existing generative AI applications such as ChatGPT is escalating rapidly, altering the traditional technology adoption curve.
McKinsey projects that if generative AI permeates all industrial societies, the economic value generated could amount to an astounding $6.1 trillion to $7.9 trillion annually.(*1)This would have a monumental impact, generating value equivalent to the GDP of a moderately developed G7 nation.
In such scenarios, the potential value of generative AI becomes apparent through its implementation in various use cases. End users, such as businesses, are directly involved in the value creation process through these use cases. Consequently, the degree to which this potential value can be maximized hinges on the development of appealing use cases.
Contents
- Generative AI value creation process and value chain
- Exploring how to adopt a foundation model from an end-user perspective
- Choosing and developing the right use cases
- KPIs for Generative AI and effective approaches to prevent use case failures
- Unlocking the power of Generative AI: three recommendations for executives
Generative AI value creation process and value chain
Generative AI value creation process encompasses the development of infrastructure, the preparation of extensive datasets for pre-training, the creation of foundation models to augment generative AI, and the construction of applications or use cases that harness the potential abilities of foundation models for tangible value (See Figure). The form this value creation process takes varies based on stakeholder involvement.
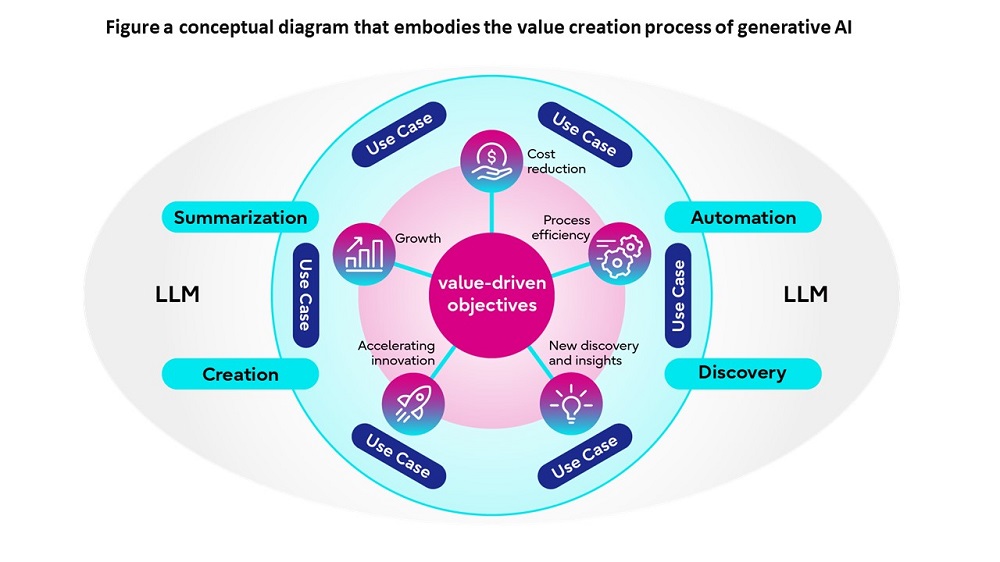
Created by author
Furthermore, the capacity of the foundation model to serve as a driving force for generative AI can be interpreted in multiple ways. However, we distill it into four primary capabilities at the level of human intellectual tasks:
(1) Automation: This fundamental level includes data collection, organization, and basic calculations.
(2) Summarization: The subsequent level necessitates the ability to distill vast amounts of information and highlight key points, requiring comprehension and recontextualization of information.
(3) Creativity: A more advanced level involves the ability to generate novel ideas and concepts, necessitating creativity and insight to produce new information from existing data.
(4) Exploration: The apex level requires profound understanding and analysis, including the ability to uncover new patterns and correlations within existing data.
Exploring how to adopt a foundation model from an end-user perspective
There are three ways to leverage the capabilities of the foundation model.
(1) Adopting an off-the-shelf model
From a user's viewpoint, you can use numerous commercially available, general-purpose applications that integrate with the foundation model, such as ChatGPT, GitHub Copilot, and Adobe Firefly.
End users, including companies with some technical skills, can also utilize pre-packaged models via APIs provided by foundation model vendors. Through prompt engineering techniques, which involve creating user-generated prompts for questions and instructions to obtain the desired output from the AI model, you can enhance performance for your use cases. Prompt engineering can be performed without the need to retrain the model or alter the number of parameters or other structures.
(2) Customized practices that integrate off-the-shelf models with your data
On the other hand, enterprise users aiming to maximize the value of their own data or meet specific requirements must not only develop compelling applications but also select the appropriate foundation model and customize the model.
Customization also involves diverging from the original model by using your own data to fine-tune the existing model for a specific domain or task. Fine-tuning typically requires a substantial amount of data and computational resources to adjust the model's parameters.
(3) Developing a foundation model tailored to your company's objectives
Certain prominent companies, particularly those in the technology sector, have developed their own foundation models. However, constructing a foundation model necessitates substantial financial resources, extensive data, deep expertise, and a robust computing infrastructure.
Thus, the adoption strategy depends on technical capabilities, financial resources, cost-effectiveness, model diversity, and deployment speed.
Choosing and developing the right use cases
As generative AI foundation models and related technologies rapidly evolve and diversify, users are increasingly interested in the emergence and development of compelling use cases. In fact, many use cases for generative AI have been proposed or implemented. For example, McKinsey estimated the potential economic impact of generative AI by analyzing 63 possible organizational use cases based on the initial impact of generative AI technology since its introduction.(*2)
Therefore, there are two approaches to deploying generative AI to achieve value creation through business transformation. One way is to focus on using off-the-shelf models and applications for easy profit. Because value creation through generative AI requires innovation in working and business processes, early results can drive acceptance within an organization and increase momentum to expand innovation. One approach is to use customized models and applications based on your organization's data to restructure your business, customer engagement, and products and services.
In both approaches, a value-driven mindset is critical and key to defining the business case and achieving success. At the same time, you need to select and develop priority use cases that can derive the most value from generative AI.
(*2) McKinsey (June 2023) The economic potential of generative AI: The next productivity frontier
KPIs for Generative AI and effective approaches to prevent use case failures
With the end user making the investment, you need to prove the business value generated through the use case. Fortunately, the research on KPIs to evaluate the success of generative AI has deepened. As an example, the report released by the Google Cloud lists 12 KPIs (*3). For companies, how you measure metrics and set benchmarks is critical to evaluating the success of your KPIs and should be based on specific business goals, industry best practices, and past performance.
However, just like any other emerging technology implementation there are many instances where companies are over-immersed in generative AI use cases from a technology perspective and fail as a result.
To mitigate the risk of use case failures, we think it would be effective to take the following steps.
(1) Clarification of business objectives
First, set clear business goals for what you want to achieve. All executives should consider the goal of implementing Generative AI to transform existing business models, shape new business models, and create new sources of value.
(2) understanding of technology
To realize your business goals, you need to understand what problems the generative AI you're deploying can solve, what value it can provide, or what it can't or won't solve.
(3) Identifying your priority use cases
Select specific use cases for fit for purpose based on your business objectives and technical understanding.
(4) Prototyping and Validation
Build prototypes for selected use cases and verify that they contribute to achieving business objectives. This validation should include the Proof of Concept and Proof of Business.
(5) Scale Up
Once the validation is successful, the use case is fully deployed and scaled up in the same domain to increase value creation. You need also work in parallel to develop new relevant use cases from validated use cases.
(*3) Google (July 2023) The executive’s guide to generative AI
Unlocking the power of Generative AI: three recommendations for executives
Based on the results of the above research and insights, we would like to make the following three recommendations.
Recommendation 1
Redefining business strategy: Setting value-driven goals and upgrading DX plans
From a management perspective, generative AI is a tool for value creation, and you need to choose the best tool for your purpose. To do this, it is important to set a value target for the entire company first.
And it is important to design generative AI as an integral part of business solutions, such as digital transformation (DX) solutions, to achieve management objectives. Generative AI should not be seen as a stand-alone plan that focuses solely on task automation within the enterprise, but rather as a “Enterprise DX+ Generative AI” plan that is integrated into the entire enterprise DX strategy.
Recommendation 2
Developing hybrid use cases leveraging the synergy of Generative AI and traditional AI
There are a variety of tasks in corporate activities, including verbal, nonverbal, or mixed tasks. but with advances in technologies such as image recognition and code generation, generative AI is increasingly applied to non-verbal tasks.
However, generative AI methodologies that are probabilistic seek optimal performance through learning and growth, rather than relying on fixed software or algorithms. This may result in output that is not always accurate, can be unpredictable and difficult to control. From a risk avoidance perspective, companies may be reluctant to adopt generative AI. Traditional AI, on the other hand, produces predictable results based on pre-established rules and algorithms or deterministic functions set by the developer. In this context, to control the behavior of generative AI and ensure its reliability and safety, it is important to develop "hybrid use case or hybrid application" in conjunction with definitive models such as conventional AI. This means that the two types of AI can complement each other to maximize their strengths and minimize risk.
Fujitsu has already developed and implemented a hybrid use case for consumer services that integrates the existing AI "Actlyzer" with generative AI.(*4) This balanced approach, coupled with technological advances, can be expected to penetrate industries and occupations that are less affected by generative AI.
Recommendation 3
Exploring a balance between utilizing the emergence capabilities of Generative AI and achieving trustworthy AI
Beyond the conventional elements of reliable AI, we believe that 'transparency,' 'fairness,' and 'robustness' are particularly important due to the unique nature of generative AI.
For example, while generative AI has the emergence capabilities that enable new value creation, it can generate incorrect information and generate inappropriate responses to user input. The phenomenon called "Hallucinations (know-it-alls)" is a particularly important issue in utilizing generative AI. Strategies to prevent these know-it-alls include careful selection of AI learning data, rigorous monitoring of the AI learning process, ensuring that AI does not learn incorrect patterns, and mechanisms to detect and correct AI behavior when necessary.
In fact, major IT vendors are already developing such technologies. For example, Fujitsu has developed and is making available to customers hallucination-detection technology, an AI trust technology that enhances the reliability of interactive generative AI responses.(*5) The developed hallucination-detection technique can detect hallucinations more accurately than existing methods.
Therefore, certain constraints must be imposed to regulate the behavior of generative AI and ensure its reliability and safety. However, the imposition of such constraints may suppress the new capabilities of generative AI to some extent. This is one of the trade-offs when using generative AI. In the future, as the understanding and control of AI advances with the advancement of technology, it is expected that the use of generative AI with a higher degree of freedom will become possible.
For more information, see Fujitsu's insight paper Generative AI: Use Cases as the Pathway to Value Creation
(*4) Fujitsu press release:Fujitsu deploys AI customer service solution for field trials at supermarket chain in Japan
(*5)Fujitsu press release:Fujitsu launches new technologies to protect conversational AI from hallucinations and adversarial attacks
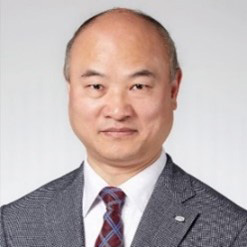
Editor's Picks
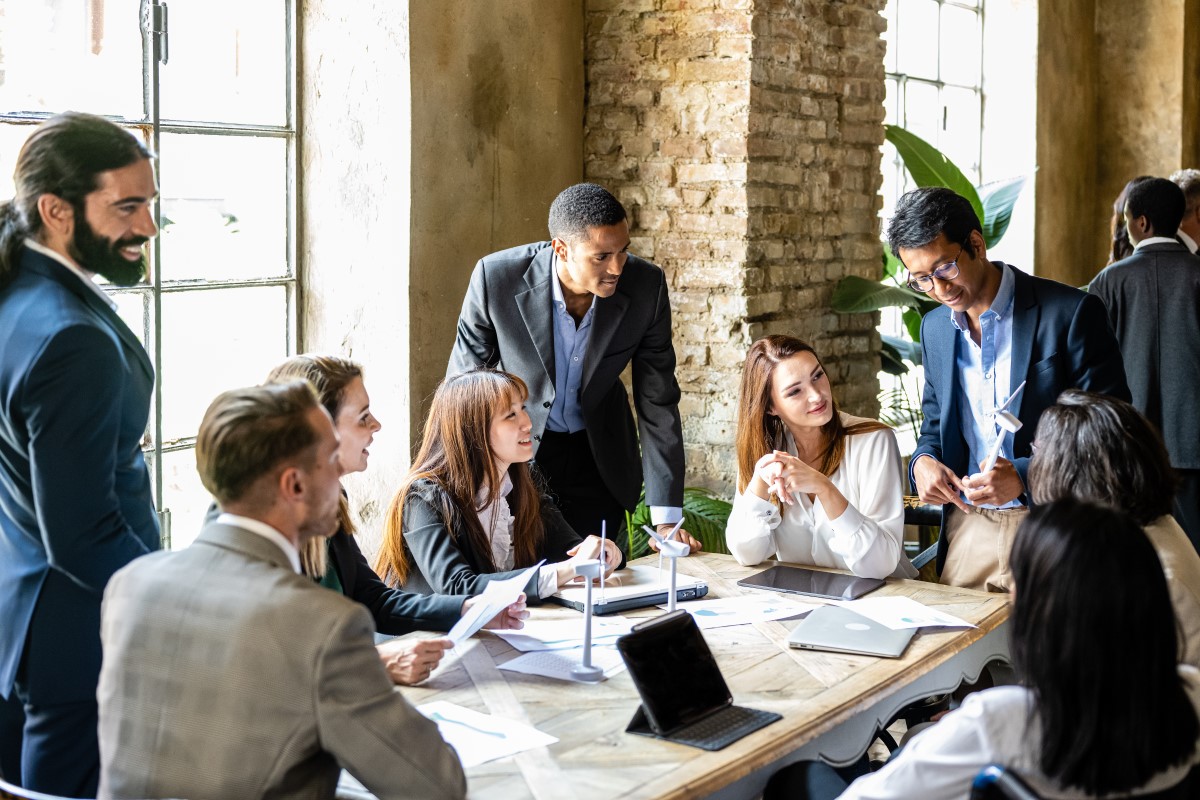
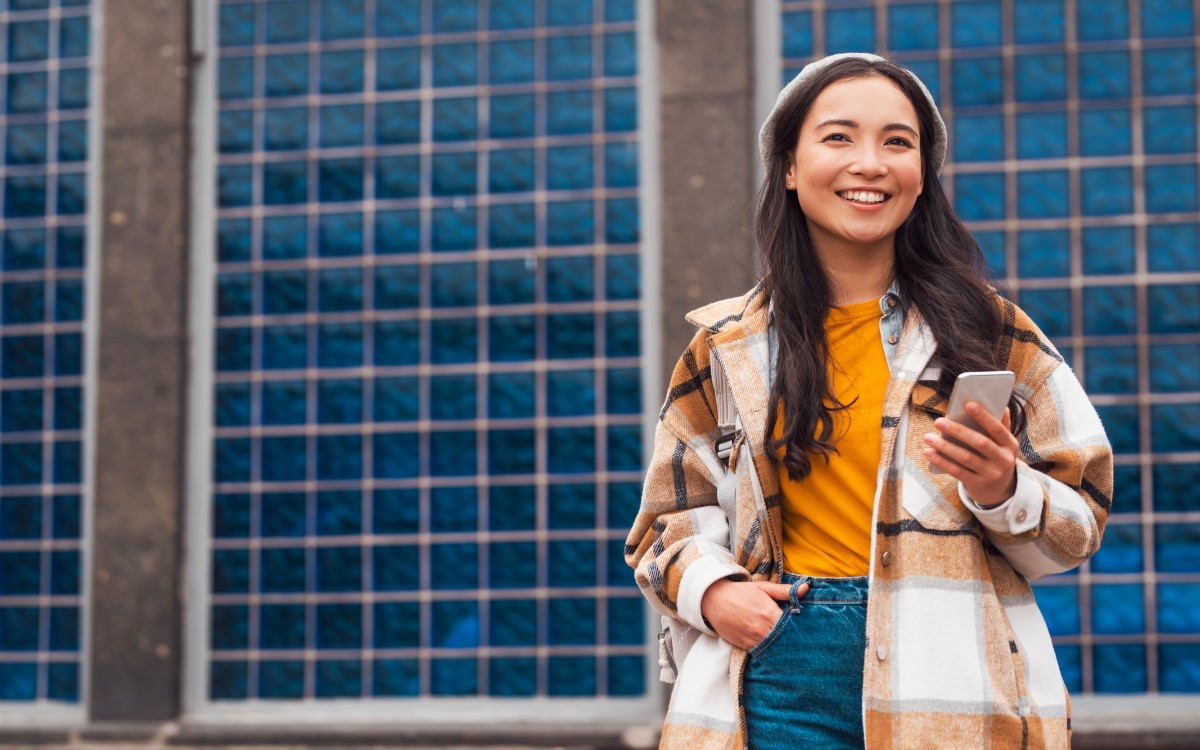