Eliminating the social consequences of damaged cardboard packaging with unaffected contents using AI [Part 2]
Fujitsu / May 18, 2022
Suntory and Fujitsu are working with the distribution industry on the first standardization of corrugated cardboard container stain and damage criteria to reduce the social losses this causes.
In the second part of the interview, we talk to Hideyuki Uemae of Suntory Holdings Limited, who initiated the project, and Takehiro Yoshida, Data Scientist, Uvance Unit at Fujitsu Limited. They used technology to respond to Uemae’s wish to involve the entire distribution industry in this unprecedented initiative to solve social issues.
Contents
- An unprecedented project that aims to solve social issues by involving the entire distribution industry
- AI and human collaboration to determine stain and damage level
- Agile, collaborative development encourages involvement of the field and in PDCA cycles
- Ongoing efforts to improve AI accuracy – how the development of a smartphone application was a turning point in the project
- Aiming to solve many social problems
An unprecedented project that aims to solve social issues by involving the entire distribution industry
— We understand that you two are the founders of this project. What made you decide to involve the distribution industry in determining packaging cardboard stains and damage?
Uemae: I oversee site improvements in the Supply Chain Management Department. Packaging corrugated containers rejected for being dirty and damaged in the distribution industry has long been a social issue, but we have been unable to find a lasting solution to the problem.
The company was aware of the above but, still, it was difficult to promote the project because the return on investment (ROI) wasn’t guaranteed, as is usually the case with system development. It was difficult to understand how to manage this because the on-site situation is not documented as data.
When we talked to Fujitsu about this issue, they agreed that they could create a solution using AI, and we began to work on a specific project.
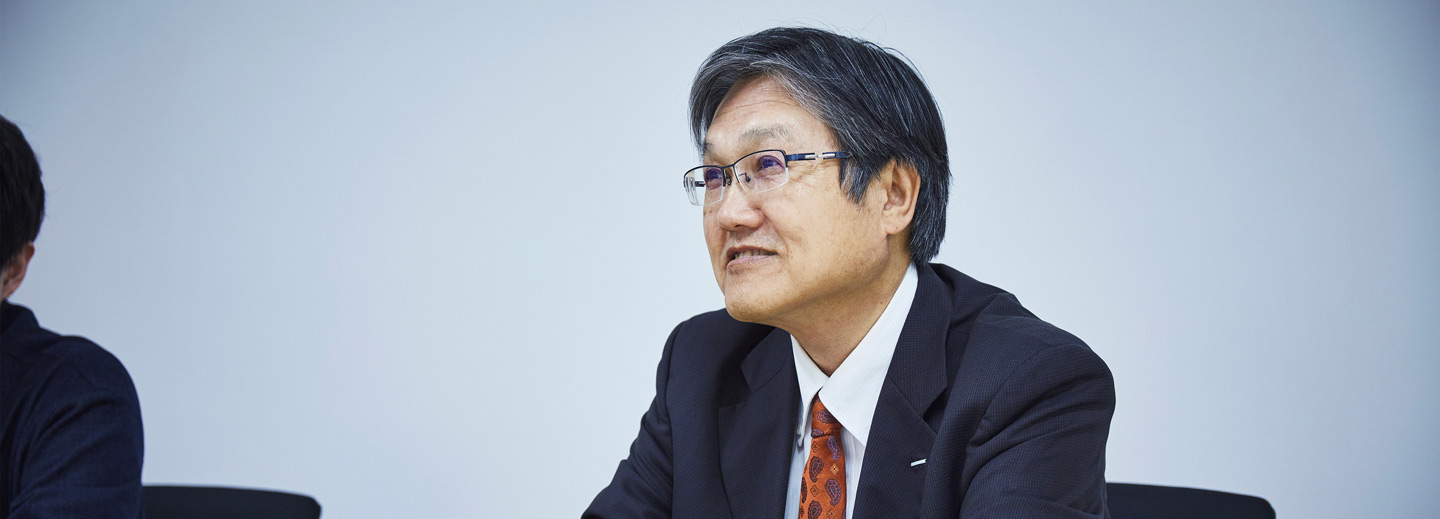
Yoshida: As a data scientist, I am interested in solving social issues with AI, and this project was right in line with that. However, the reality in Japan is that investment in AI projects is extremely low due to ROI concerns. Considering the financial loss of returns due to cardboard stain damage, development costs of tens of millions of yen or more should be available.
— How did you break through that barrier?
Yoshida: The whole distribution supply chain should mitigate social loss from stained and damaged cardboard packaging containers. Therefore, we began by creating a vision for the entire ecosystem.
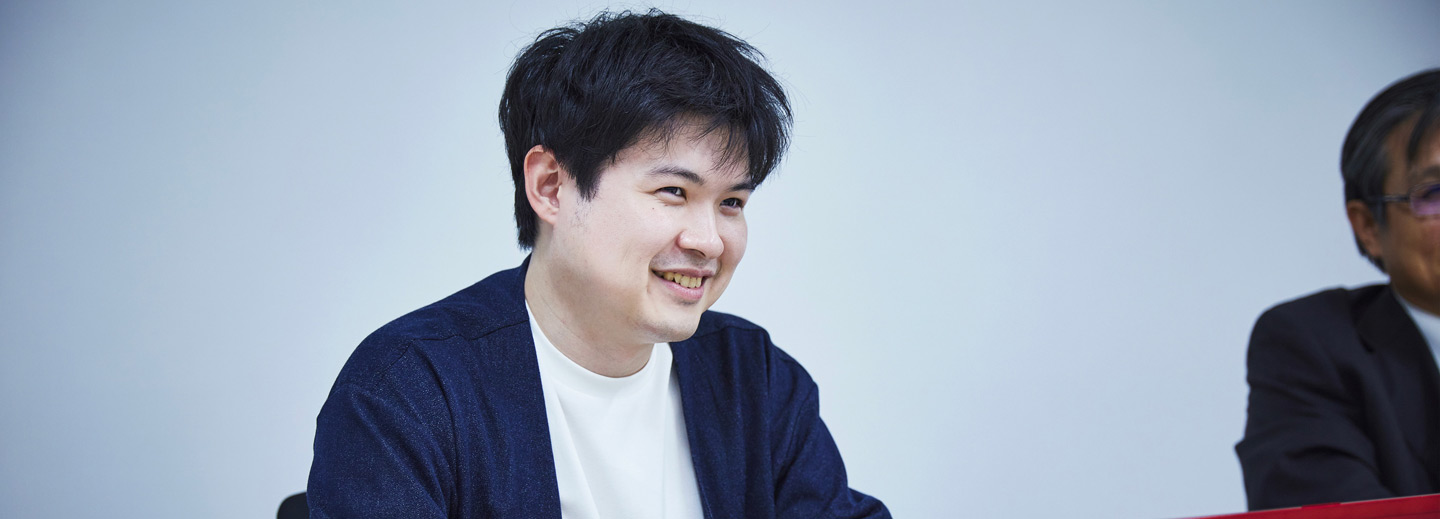
Uemae: Specifically, overall project concept is that “we are going to create a completely new mechanism that will lead to DX for the entire distribution business”. We will standardize corrugated cardboard stains and damage and unify people’s opinions in the distribution industry by collecting and storing data along with associated situations. Because we were creating an unprecedented mechanism, we formed a joint development with Fujitsu rather than a client-developer relationship.
Determining stain damage to packaging corrugated cardboard containers also involves Japan’s unique culture and sense of beauty. Fujitsu is an inspiring partner because of its knowledge of the domestic supply chain situation and SDG initiatives as well as those Japanese cultures, plus its ability to involve the entire industry.
Yoshida: We believe that a single company cannot complete this system. It must be spread throughout the entire distribution industry to gather lots of data. Mr. Uemae led negotiations with various organizations and companies to get the industry involved. I learned a lot from his efforts to increase the number of supporters that resulted in the company winning the Excellence Award in the Supply Chain Innovation Awards 2020 (*).
(*) The Supply Chain Innovation Awards are presented by the Council of Manufacturing, Distribution, and Sales to companies that have led the industry by making outstanding efforts to optimize the entire supply chain through cooperation with manufacturing, distribution, and sales.
AI and human collaboration to determine stain and damage level
— How exactly do you determine corrugated cardboard container stain and damage?
Yoshida: Deep learning automates whether to ship corrugated containers, a decision which on-site workers previously made.
We ask on-site personnel to photograph cardboard containers using a stain/damage assessment smartphone application. Image analysis then classifies (1) tears and damage and (2) body swelling.
By comparing the results with past data in the AI recommendation model, five similar images with past shipping availability decisions are recommended. However, due to variations in the decisions of previous personnel, say, three of the recommendations may be indicated as acceptable for shipment, and the remaining two may not be. In such cases, the on-site person in charge selects a recommended reference image that is most like the photo taken and decides to accept the shipment on that basis.
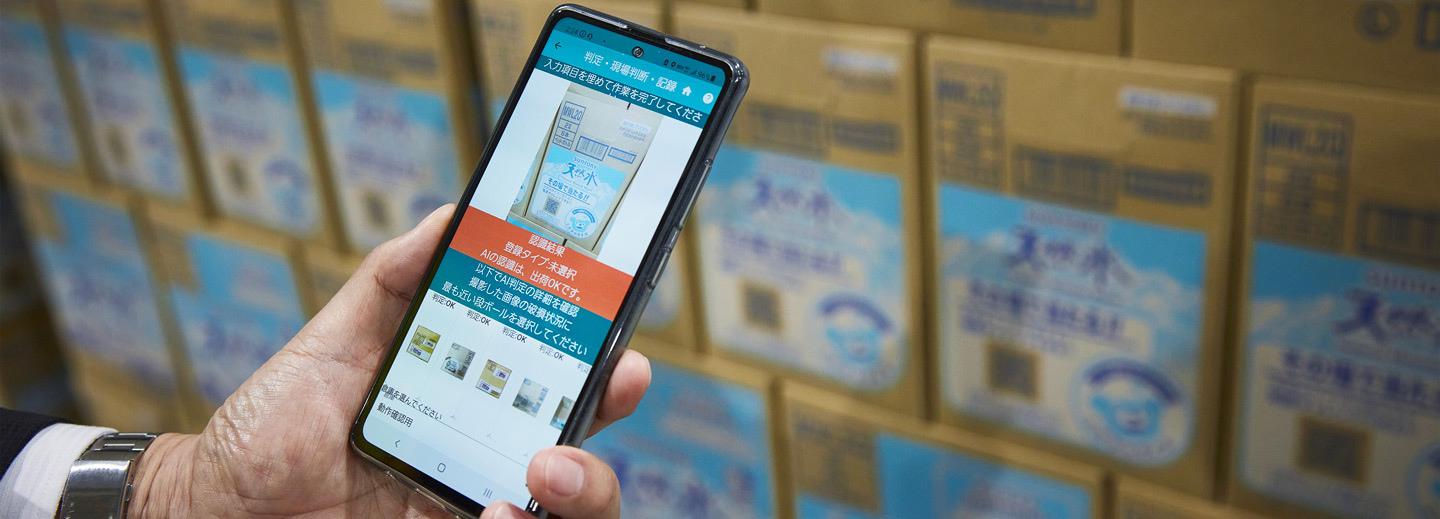
— So, you are not relying solely on AI judgments, but adding human judgment as well?
Uemae: Since AI judgments are based on past human judgments, if there are too many strict judgments before, judgments by AI tend to be more severe. Therefore, we have ensured that AI criteria are continually updated with current human decisions.
Yoshida: In addition to images of damaged corrugated containers, business records related to shipping can also be accumulated, making it possible to visualize problems faced on site. Even if the judgment process is eventually fully automated, we still need to accumulate as much judgment data as possible. We made a system in which AI and humans cooperate to make decisions because it also motivates active judgment data input.
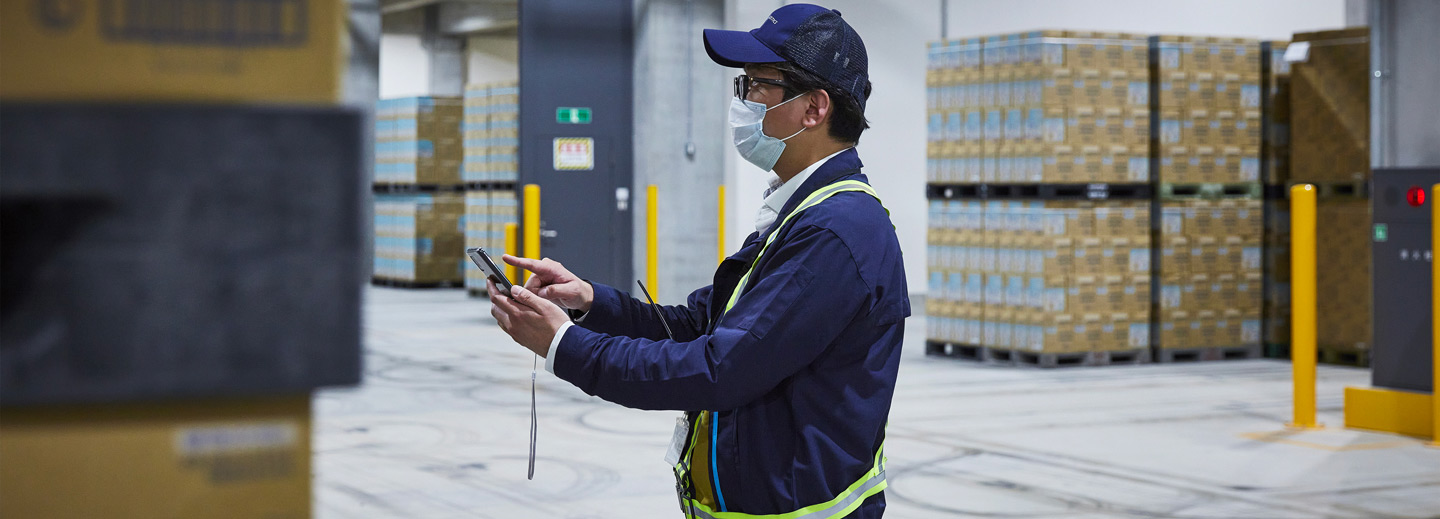
Agile, collaborative development encourages involvement of the field and in PDCA cycles
— What kind of development approach did you take to create this unprecedented system?
Uemae: We based the development on the premise that the Plan, Do, Check and Act (PDCA) cycle would be objective for all parties involved. We converted on-site conditions into data and received requests from various perspectives, such as differences in judgment criteria among manufacturers and distributors, so it was necessary to create an unprecedented mechanism to handle this. We adopted a venture-like and agile development approach so that the system proceeds by sequentially adding opinions on both the development and the user sides. Ideas, developing, testing, and improving the system can all happen very quickly.
Yoshida: We created prototype applications so as not to slow down the PDCA cycle and quickly improved them while observing their use on site. Complaints from the site also helped us improve the workflow design and the usability of the application generally.
Uemae: [Laughing] I was surprised when what I requested at the beginning of the meeting was already implemented by the end! Close communication with Mr. Yoshida was essential, so we communicated frequently using chat app to share ideas with each other and making improvements. We also reduced the testing period by conducting system testing on the user side. I believe that these agile improvements ultimately led to increased satisfaction from the system site and convinced new advocates in the industry.
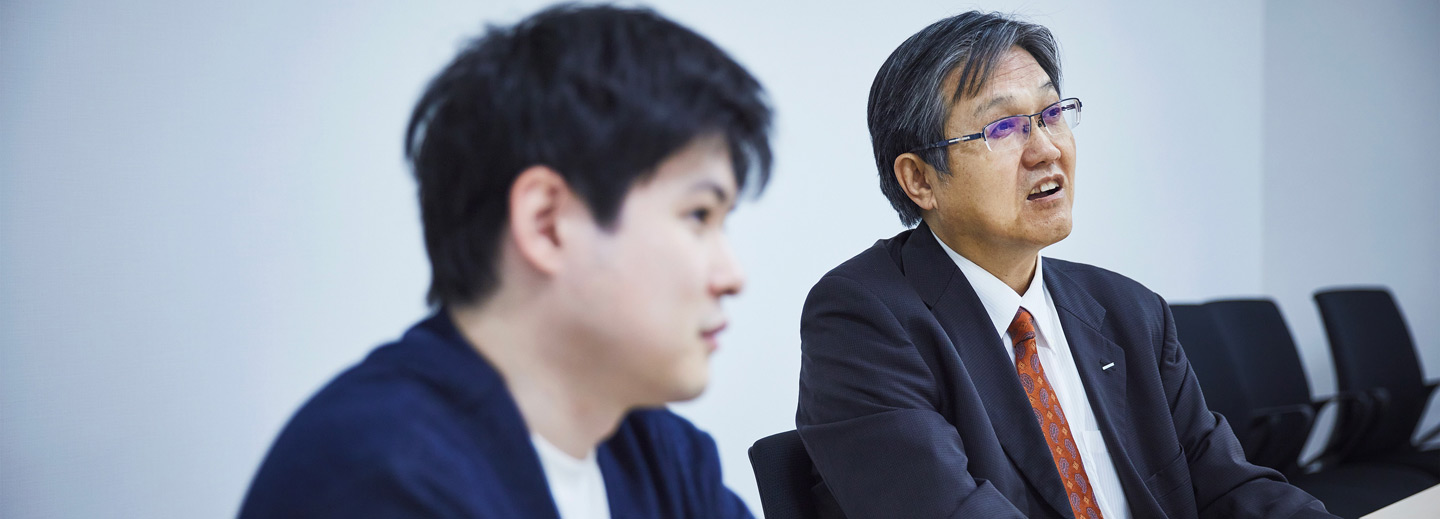
Ongoing efforts to improve AI accuracy – how the development of a smartphone application was a turning point in the project
Did you face any problems in this very unusual solution development?
Uemae: Many sample photos are needed to train the AI to improve accuracy. In the beginning, we collected individual photo samples on a PC and managed to verify AI models by taking the machine to the site or having the photos sent from the site. That process was not an agile way to make improvements, and discussions were not progressing, so we solved the problem by switching to the current smartphone application.
Yoshida: In demonstration experiments, it is common to undertake small-scale work on a PC, but they are not always available, plus they are heavy to move. We decided to take the plunge and create a smartphone application. This strategy changed the situation drastically, creating an environment where each person could use their smartphone as needed to grasp how the workflow would change.
— You are currently in the phase of improving the accuracy of cardboard stain and damage determination. What exactly are you doing about it?
Uemae: We are in the process of finalizing both ‘damage type accuracy’ where the AI identifies the type of stain damage, and ‘selection accuracy’ which looks at the percentage of photos within the five judgment recommendations. Efforts are also being made to improve accuracy in the field, for example, by annotating the stained, damaged areas on the photographed cardboard with comments.
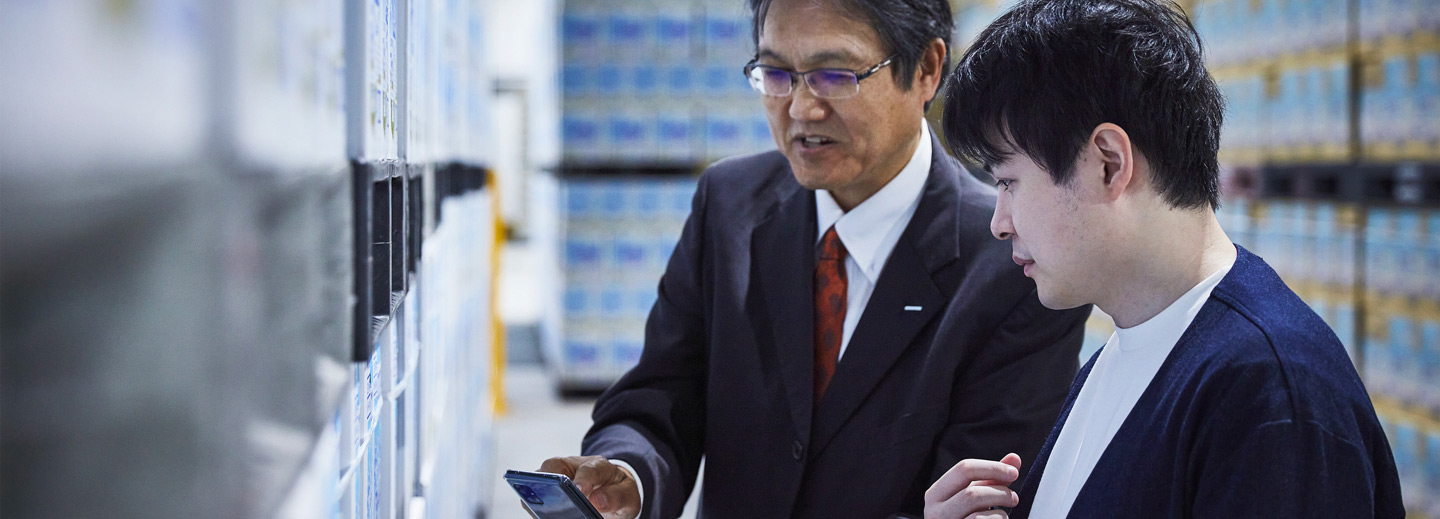
Aiming to solve many social problems
— What is your vision for the future of this project?
Yoshida: We have about 1,000 photos of stained and damaged samples collected now, but we hope to create a model for automatic inspection when we have about 5,000 samples. By that time, we will be able to advance our discussions and determine factors such as whether the position of same size hole damage impacts the acceptability of shipment. Furthermore, we would like to display statistical information on the application dashboard relating to the time of year and situation in which certain decisions were made, and provide advice on how to place the product so that stain damage is less likely.
Uemae: The balance between AI and human judgment is so delicate that even a new warehouse creates different judgments by on-site personnel, leading to lower accuracy. That is why we believe it is important for the entire distribution industry to cooperate and follow certain judgment criteria. We are developing the system so that AI and people can recognize each other – like in good human relationships – and we hope to achieve a judgment that everyone can agree on as soon as possible.
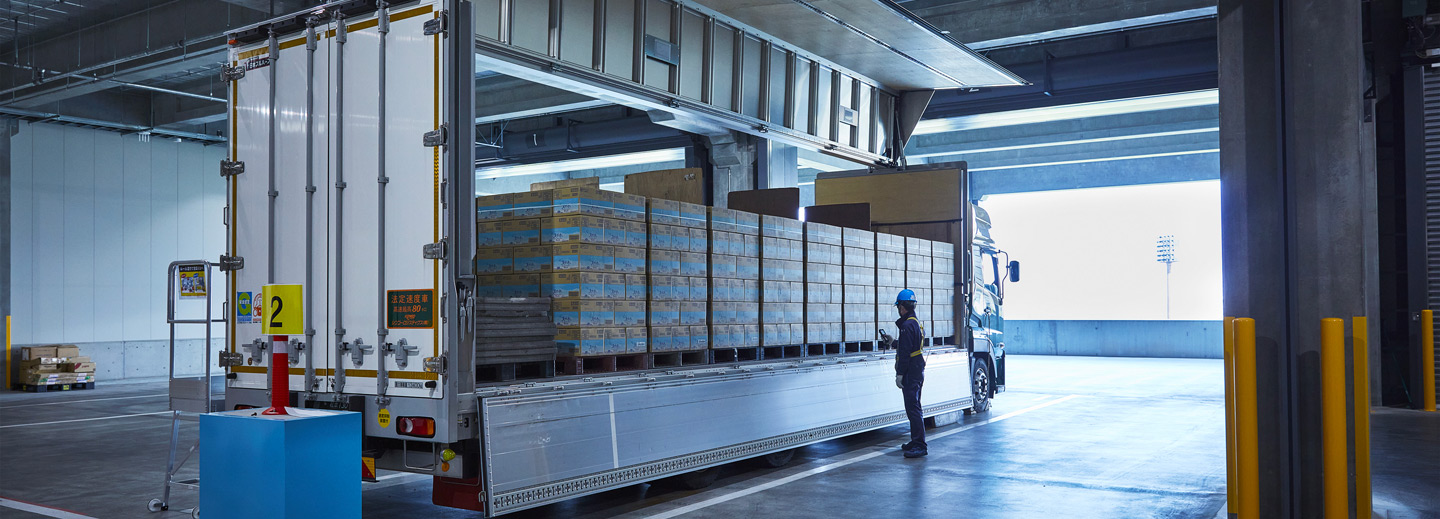
— Finally, please tell us your thoughts on the future relationship between technology and society.
Yoshida: According to the theory of ambidextrous management, to survive, a company needs to “search for knowledge” to find the target theme or solution method and “deepen knowledge” to improve the existing business. In Japan, investment in this search is sometimes neglected because of the strong demand to establish a basis for ROI. A draft, or base, for drawing up the rationale is necessary to solve this problem. We, therefore, believe that stable smartphone apps and similar technologies will enable a quick and broad overview of the entire ecosystem. A solution to various social issues will then be found at a limited cost and allow us to see beyond ROI. This work will clarify how AI interacts with associated people and applications and transform it from an AI project that replaces people into a workflow innovation project. It is crucial to proceed in a way that allows everyone to feel confident about ROI and move forward with a full-scale investment.
Uemae: The improvement of ambiguous judgments by AI also means fewer disputes between workers, which will lead to increased confidence in distributors. However, industry-wide cooperation is essential to maximize knowledge known only in the field and develop solutions. We hope this project, which used co-creation to overcome barriers, including cost, will set a precedent for solving various social issues.
Problems are not limited to dirty and damaged beverage packaging cardboard containers. There are several issues in the distribution industry that need resolving, such as safety management in forklift operations. In addition, we expect this initiative to apply to problems faced by other industries.
Ultimately, when considering the SDGs, I dream of a day when society will reevaluate the role of packing cardboard and tolerate minor stains and damage that does not interfere with transportation and use. When that time comes, AI-based judgment solutions for packaging will have come to a natural end.
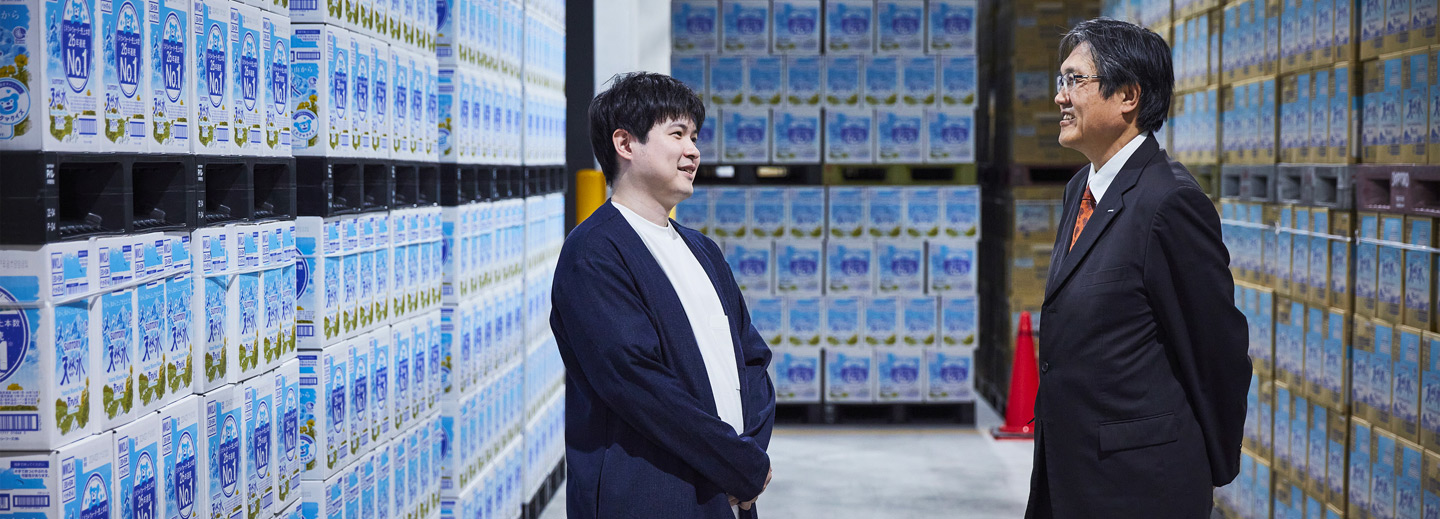
Go to article [Part 1].
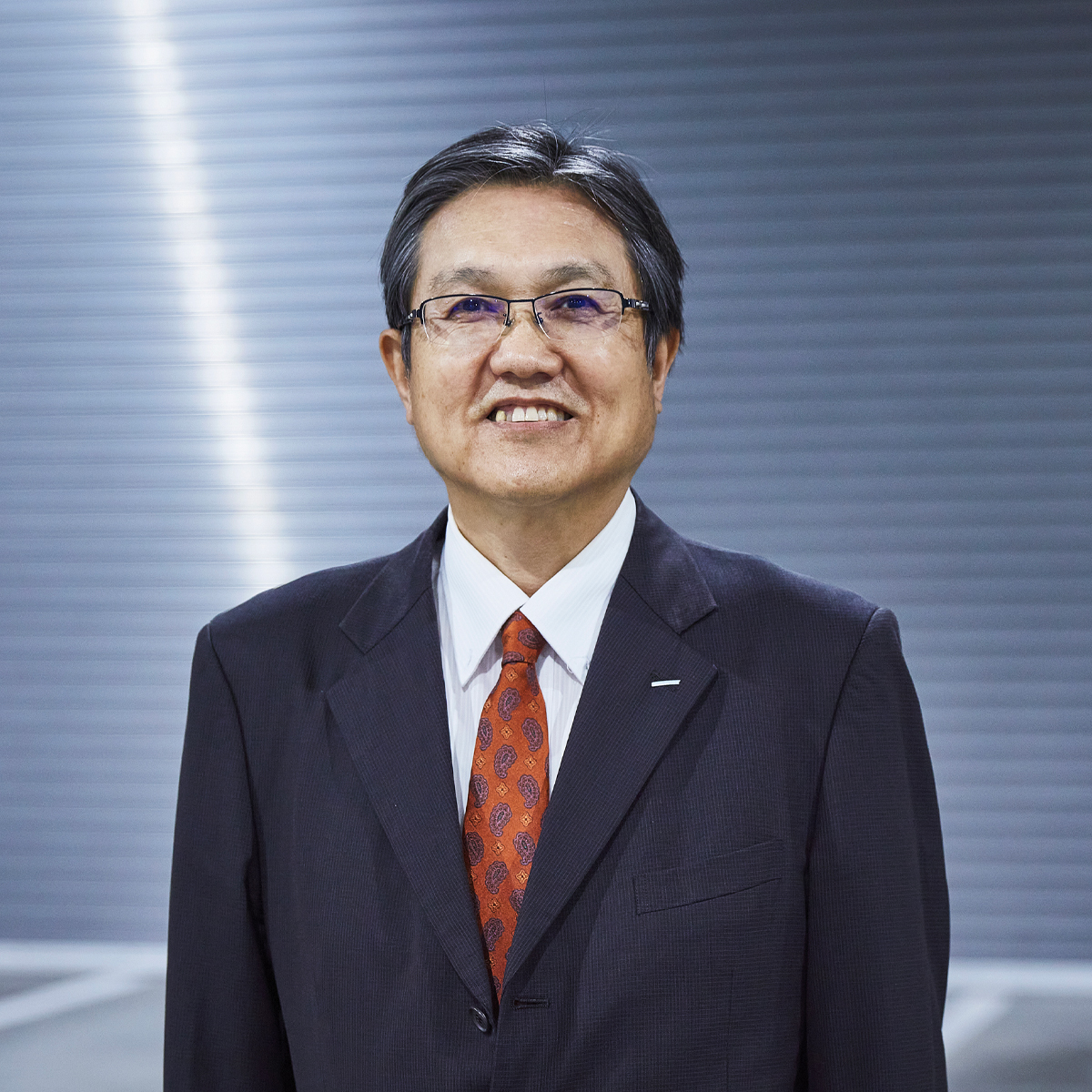
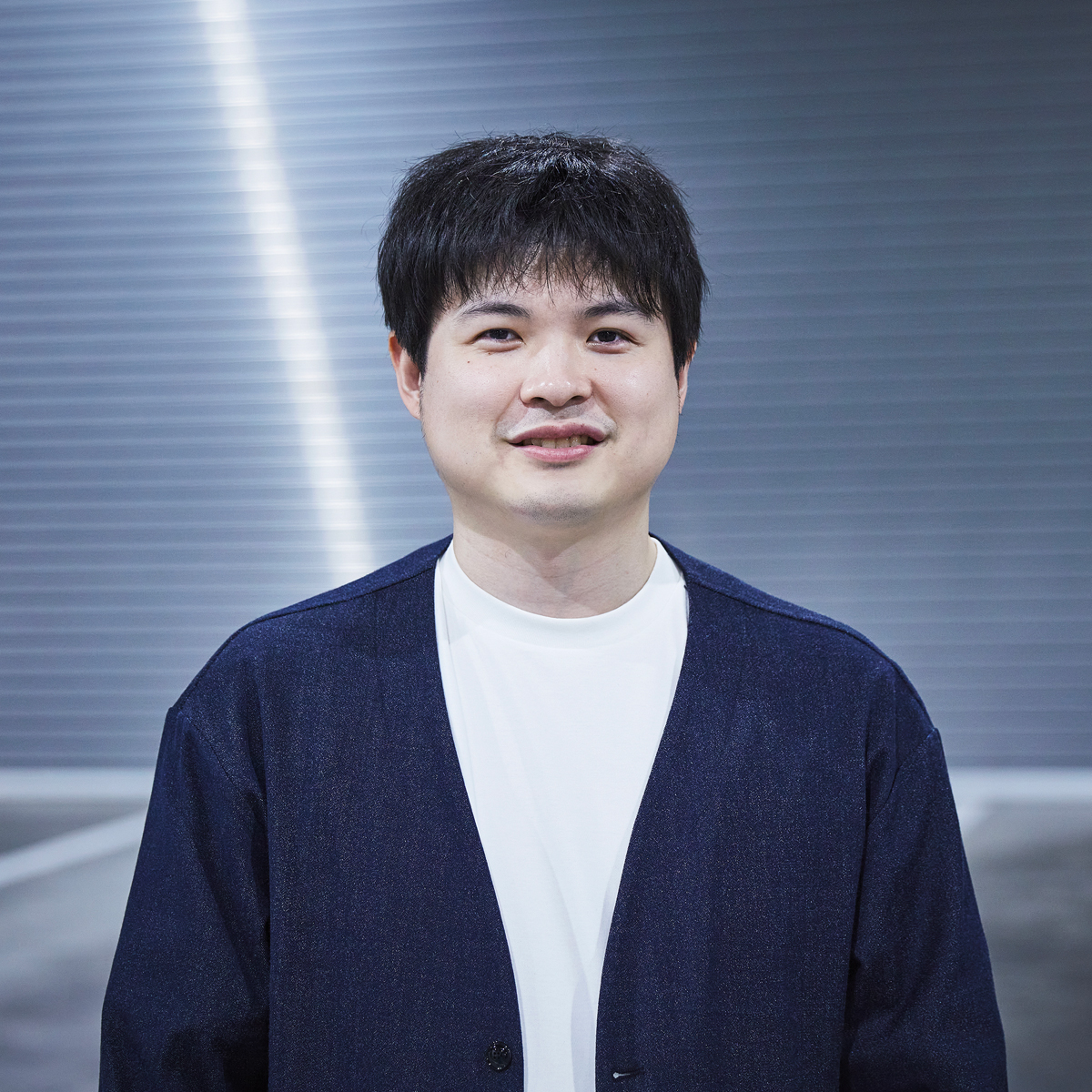
Related information
Editor's Picks
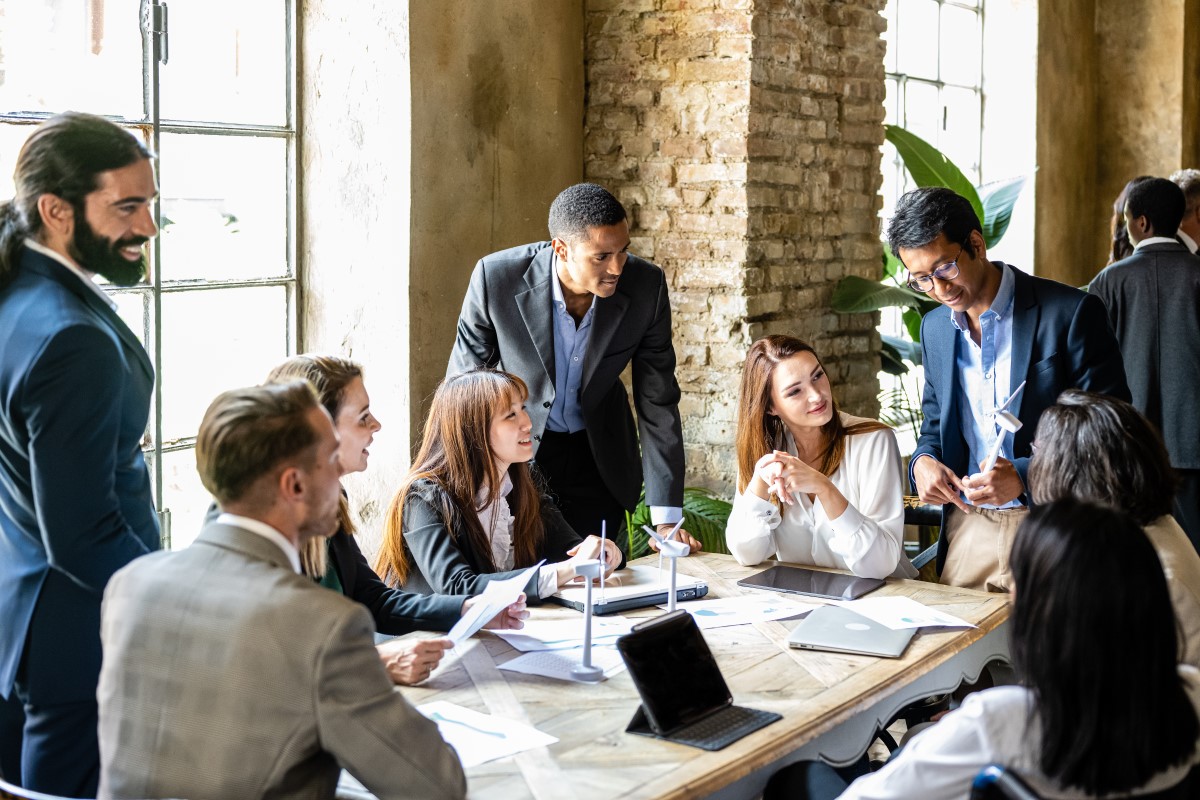
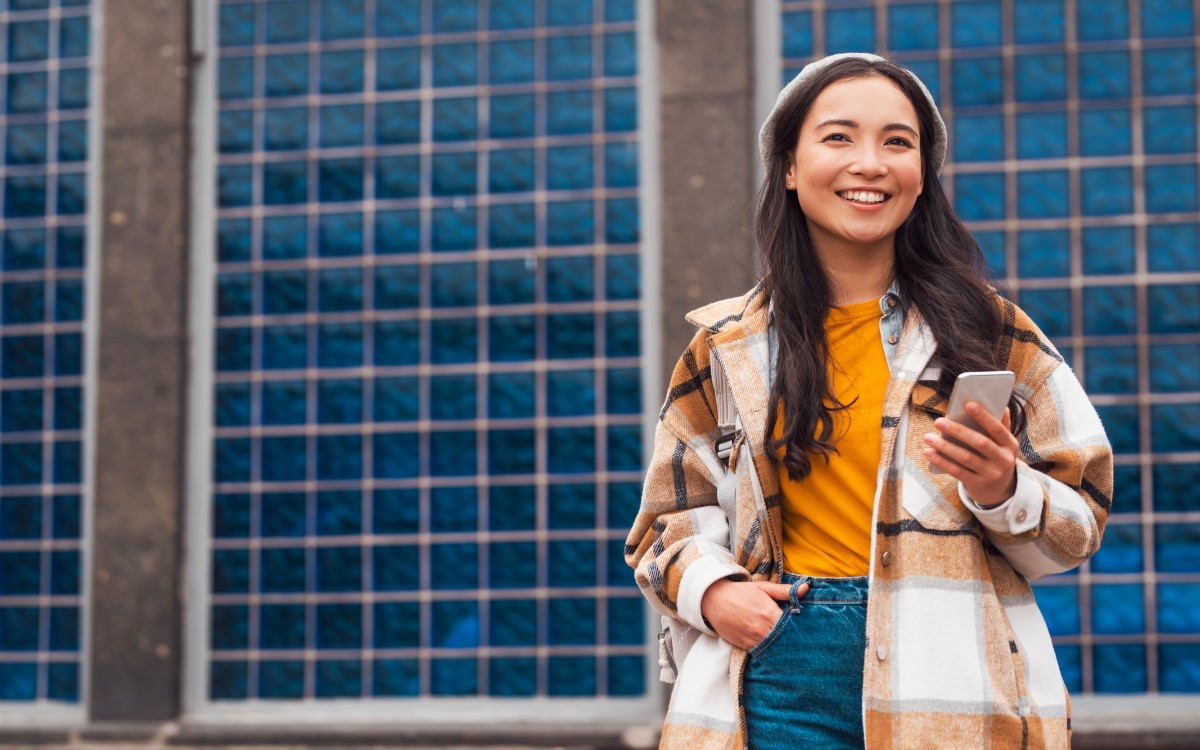