How to empower your employees to accelerate AI’s impact on your business and society? We have the answer!
Fujitsu / March 23, 2022
Few people doubt anymore that AI is going to transform business and society. But still, why are over seven out of ten companies struggling to get AI out of experimentation and into production? Oscar Jarabo, International Portfolio Manager, Data Intelligence at Fujitsu, and Albert Mercadal Playa, Global Head of Data Intelligence at Fujitsu, believe that creating MLOps factories is key to breaking through the AI logjam and empower employees to accelerate AI’s impact on business and progress towards a trusted society.
Companies are still struggling to embed AI into the business
Data-driven intelligent decisions depend on interpreting and manipulating data at a scale that is beyond human capacity. This is where AI plays a key role. The challenge is that when companies start experimenting with AI, they realize that it’s not easy. They can experiment fairly readily with two or three models, but how practical is it to scale to 20 or 30 use cases in different areas of the business? When companies try to apply AI to a companywide context then, the real logjam appears.
Difficulties range from the time and effort needed to find out what works experimentally, to the complexity of continuous integration of successful AI experiments into a development lifecycle that is sensitive to changing context.
Cultural and structural changes in the organization are also needed. Companies tend to think that AI is a plug-and-play technology without considering the potential for cultural friction within the organization. AI requires the integration of new roles, technologies and processes within an existing company structure and culture. Decision making is affected too, shifting from a top-down model to employee-empowered decisions based on data and model results.
Finally, there are questions like “does the data comply with privacy regulations?” “Can we trust the results to confidently make decisions and audit them?” And “are we using the models ethically?” Following traditional approaches is unlikely to be practical and may leave organizations exposed to reputational damage or litigation.
It’s not hard to see why many AI projects get stuck for too long in the backwards and forwards of the experimental phase and have difficulty scaling to production.
This is not what is happening in a few isolated situations. It is the norm. According to Microsoft , only 12% of companies are in a “formalization” phase, with AI models integrated into the business as an active component of corporate strategy. And only 11% are in an integration phase, on the pathway to formalization by building AI into processes, products, and services. While that sort of 23% overall adoption rate puts AI firmly in the “early majority” phase of the technology adoption bell curve, it also means that around 76% of companies either have not started at all or, more probably, are stuck in interminable PoC experimentations.
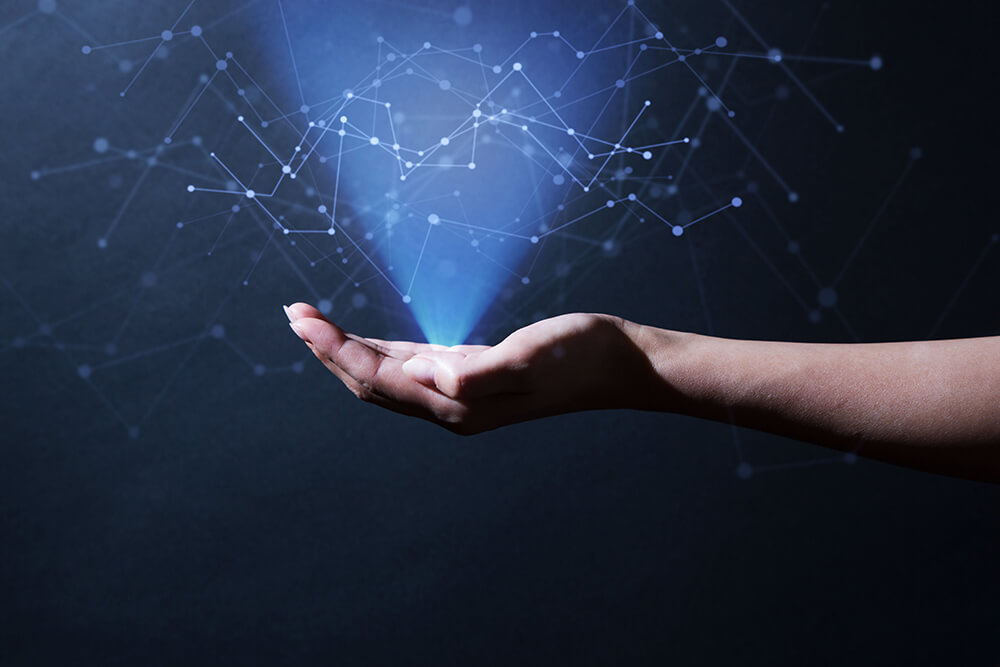
Building an MLOps factory to accelerate AI’s impact
Faced with all these demands, how can you escape from the experimentation trap and exploit the benefits of AI company-wide?
In the world of startups, incubators provide a partial solution to these challenges. They create a production line for business model development in a culture of innovation, surrounded by expertise, while spreading the risk for investors.
The same approach works for AI. We call it an “MLOps factory” to emphasize the twin aspects of agility and output efficiency that AI Dev teams need today to deliver sustainable business value. The idea is to create an end-to-end service that embeds ML (Machine Learning) as a systemic part of a business. It combines an MLOps methodology, similar to DevOps (Development Operations) but adapted to ML’s distinctive features, with technologies, cultural and structural changes, and audit capabilities, empowering people with digital power to be creative and innovative.
The first step in building your factory is to audit where you are today: What business problem are you trying to solve? Do you have the right resources, technological ecosystem, and the necessary data to have a realistic expectation of success?
The next move is to build a factory ethos that reduces production times by automating, standardizing, and optimizing processes, including continuous integration and delivery of models. This should also incorporate the systematic reuse of components.
The factory model you implement must smooth potential friction between teams with governance structures, management of cultural change, and definition of workflows. It should also help coordinate production and monitor how well models perform against their KPIs. When implemented properly, companies can gain a better grip on ML model versions and changes in the ML environment. This is important when customer behavior shifts — as it will — and you need to generate a new version of the model to reflect that.
In terms of regulatory compliance, external experts can advise on routines and tools to restrict access to personal data and ethical issues, such as unconscious bias, with the potential to cause reputational damage or litigation. The factory you are building should contain controls and processes that report how the models are used and what data is fed into the model, and allow for auditing of decisions by incorporating Explainable AI tools.
MLOps is still a new methodology and demands a diverse set of tools, processes, workflows, automation requirements, ecosystem partners and governance methodologies. Without an end-to-end MLOps factory service, most organizations will find it difficult to implement.
The MLOps factory in operation
Aligned with Fujitsu’s vision of leveraging technology towards a secure and trusted society, we are building an MLOps factory as part of the digital transformation (DX) strategy for a leading company in physical security services.
Although clear on its KPIs for using AI to improve its marketing and business operations, the company was experiencing challenges monitoring model performance and responding quickly enough when data patterns changed. Waiting six months to retrain models and get them into production was not acceptable.
In this use case Fujitsu’s MLOps factory — technically supported on public cloud or on premises — accelerates the development, training, implementation, deployment and maintenance of models. And it reduces the frictions that can arise between model development, IT, and operations teams during productization of ML models. As a result, our customer increased its capacity to deploy and productize models in its marketing and security operations, with faster speed of innovation, improved adoption, scale-up, and expansion, and more robust life cycle management.
In the same way that this company sees AI providing the future core of its operations and vision towards a secure, trusted society, a recent study conducted by Forrester for Fujitsu showed that 71% of business leaders believe organizations that fail to embrace AI risk irrelevance.
Right now, however, with more than seven out of ten businesses failing to get beyond AI PoCs, there is a complete mismatch between that DX vision and production reality. The MLOps factory is the solution to this disparity. To find out how to start building your factory reach out to us.
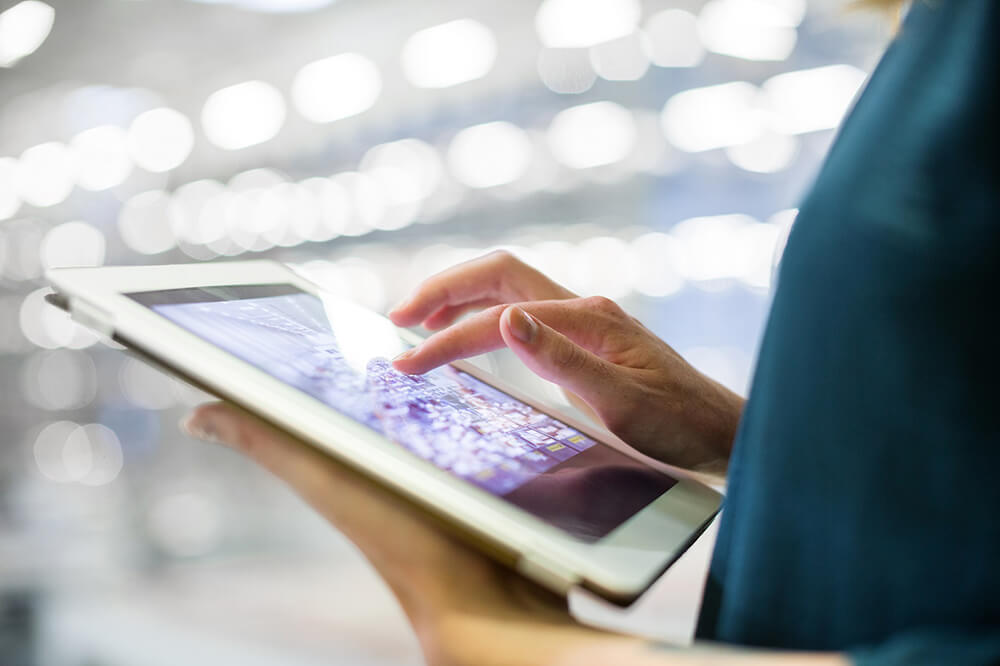
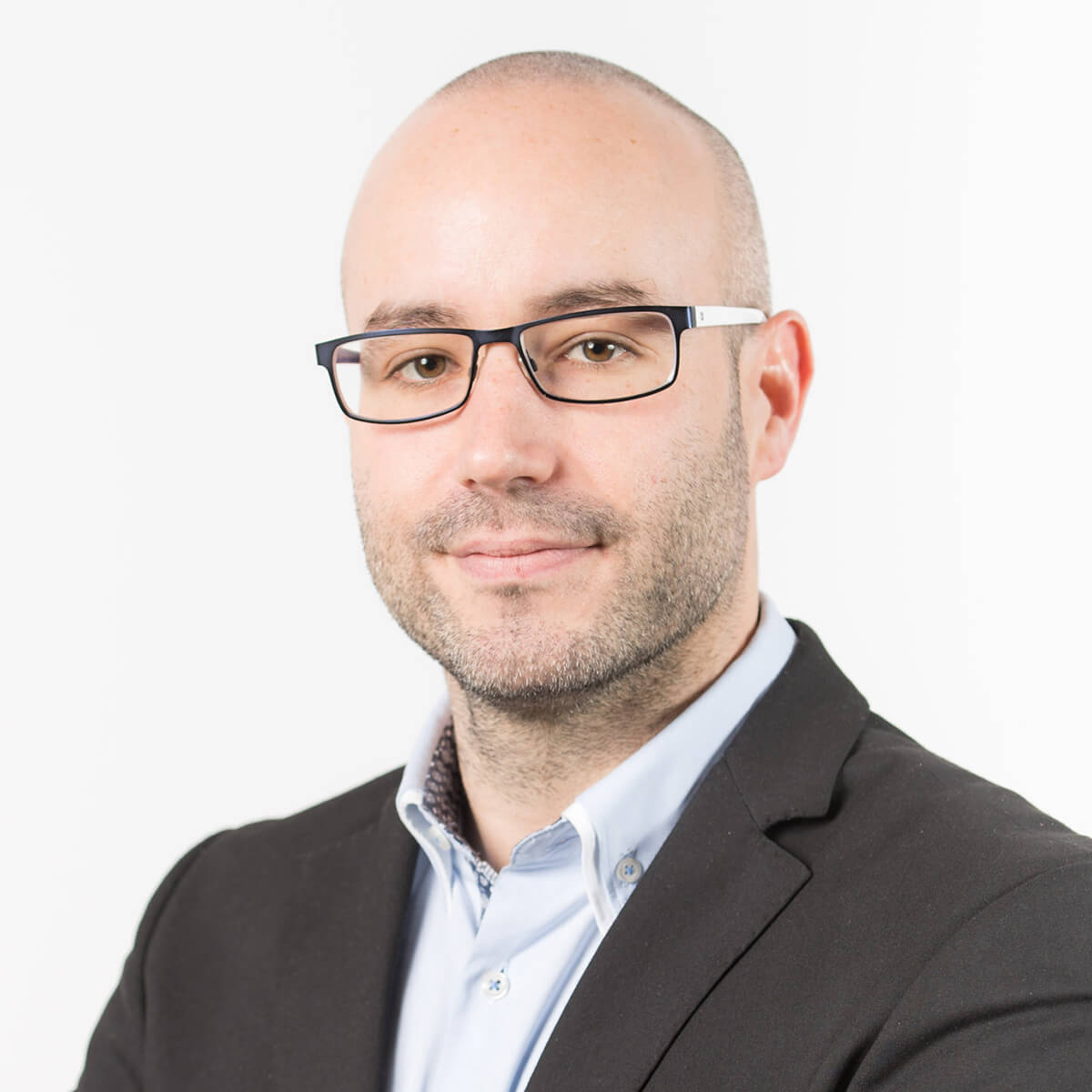
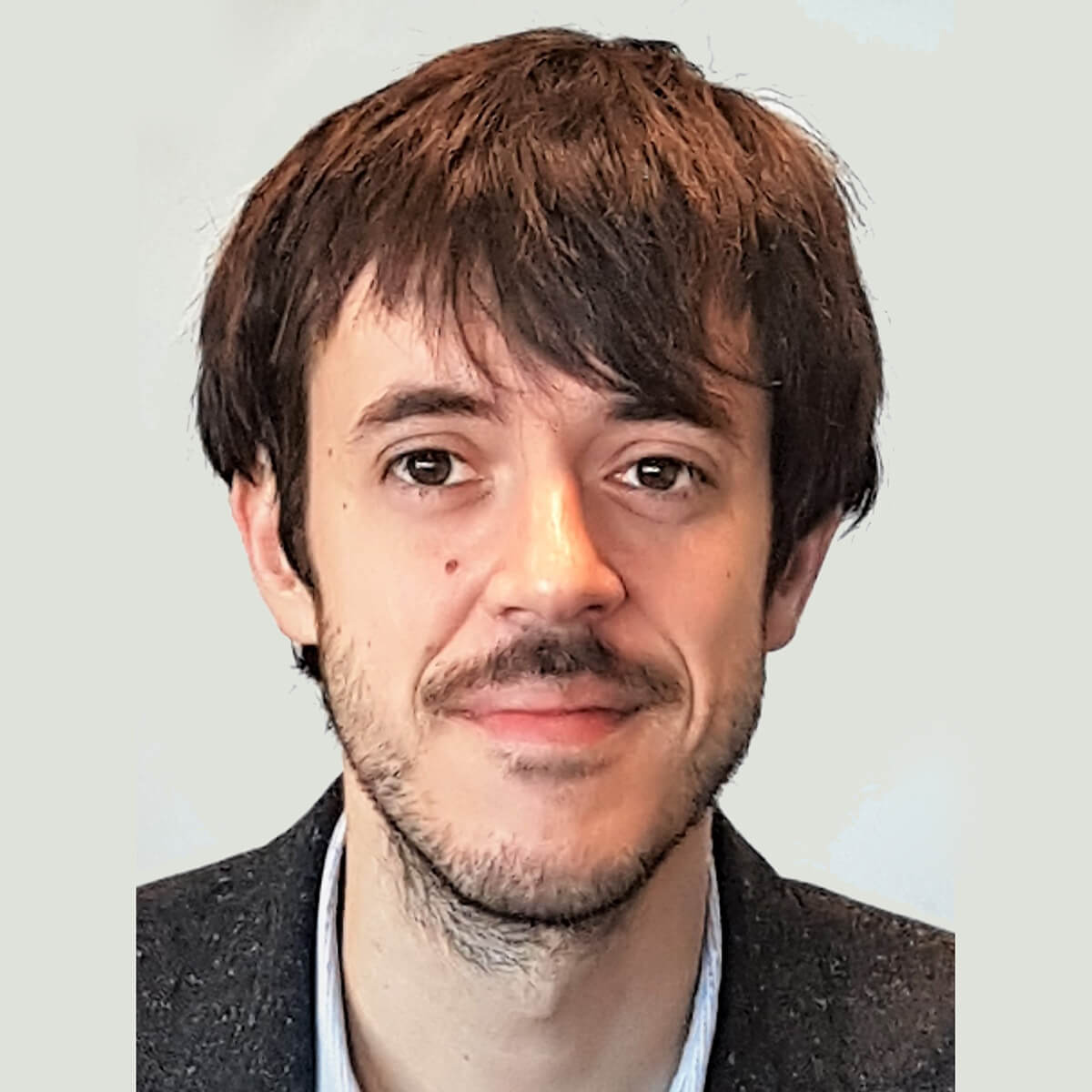
Related information
Editor's Picks
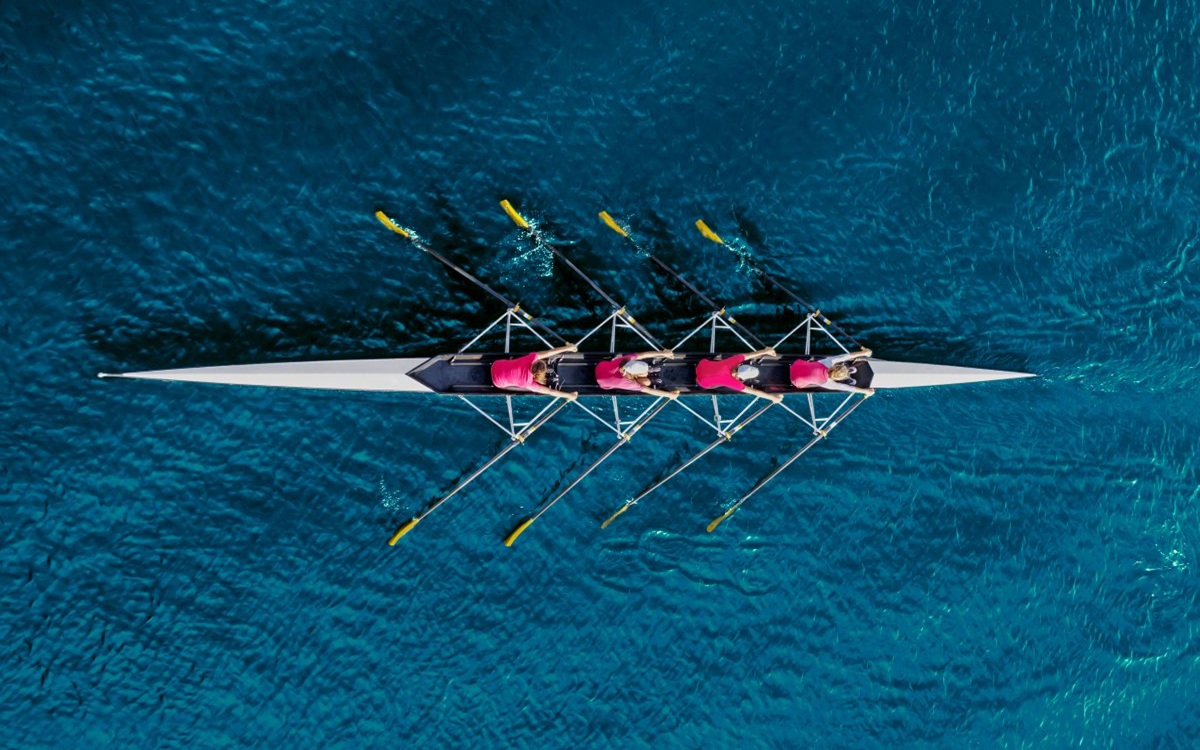
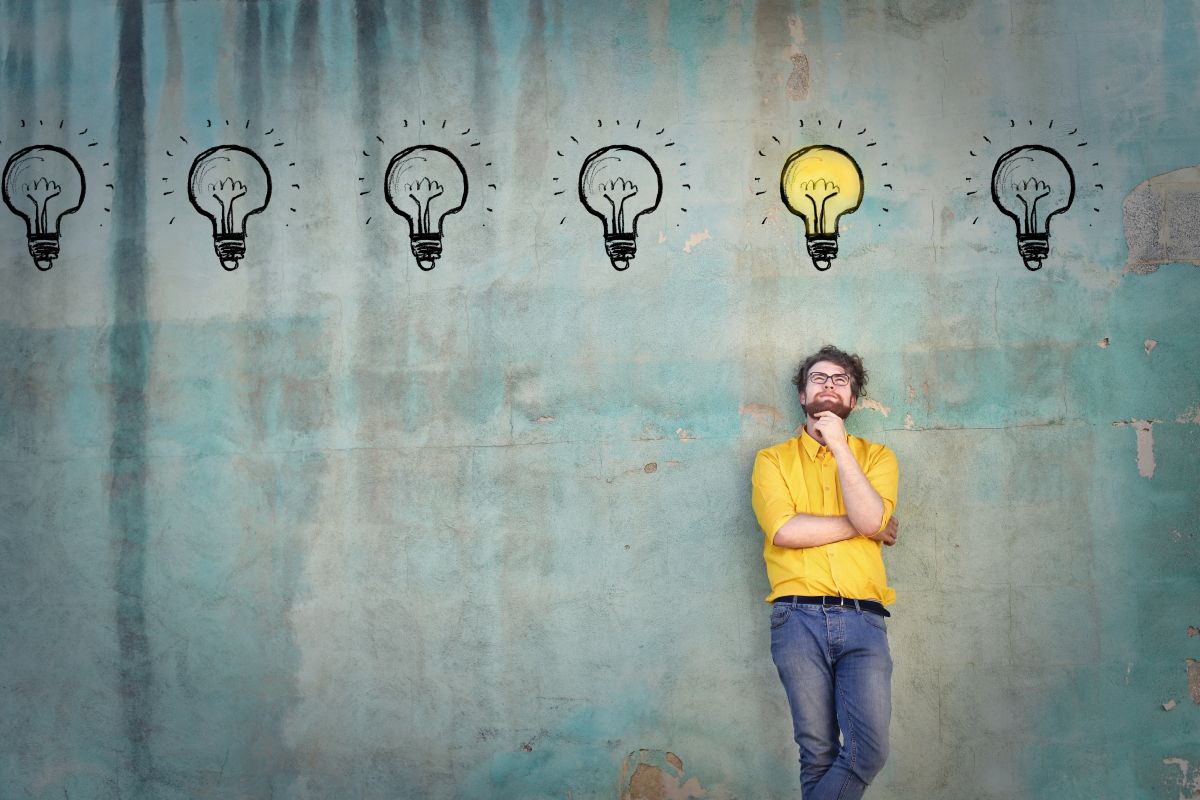
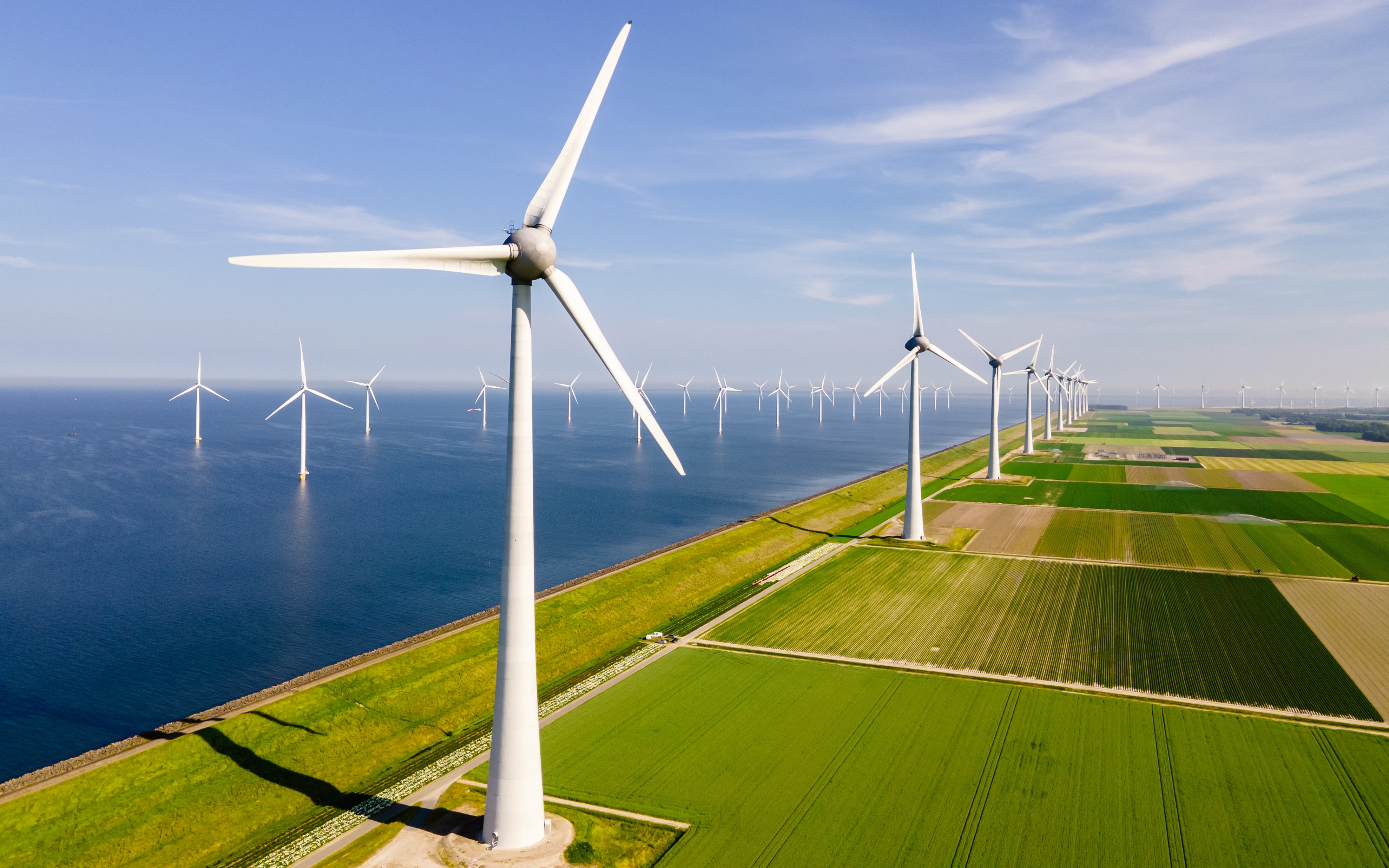